Investigating the Impact of Mobile Microlearning and Self-Regulated Learning Support on Soldiers’ Self-Efficacy and Retention within an Army Schoolhouse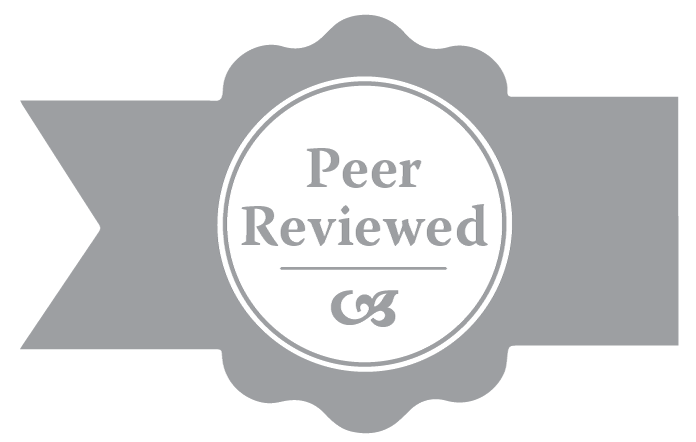
Scotty D. Craig1, Dawn L. Riddle2, Shanda Lauer3, Gregory I. Hughes4,5, Wade R. Elmore4, Chad E. Udell6, Jennifer S. Murphy7, and Laura M. Milham2
1 Human Systems Engineering, Arizona State University
2 Advanced Distributed Learning Initiative, Orlando, Florida, United States
3 Institutional Research and Assessment Division, The Army University
4 United States Army Combat Capabilities Development Command Soldier Center, Natick, Massachusetts, United States
5 Center for Applied Brain and Cognitive Sciences, Medford, Massachusetts, United States
6 Float, Morton, Illinois, United States
7 Quantum Improvements Consulting, Orlando, Florida, United States
Download the PDF 
Abstract
The current study has shown positive evidence for the adoption of the PERvasive Learning System (PERLS) into military education and training environments. A randomized control trial was implemented to evaluate the impact of PERLS within a military classroom setting. It found that PERLS use during a course could improve soldiers’ self-efficacy for content and self-regulated learning (SRL) and potentially increase completion rates for soldiers. These findings show the possibility that correctly designed technology can support users’ self-efficacy for their ability to implement SRL. Further, this study has shown that microlearning technology with SRL support, as seen in PERLS, has the potential for real-world classroom impact.
To address the training needs of the next generation of soldiers, the U.S. Army requires a systematic approach to incorporating formal and informal learning experiences into training and development. This approach is described in The U.S. Army Learning Concept for Training and Education (U.S. Department of the Army, 2017). In this doctrine, Army training leadership describes an adaptive, personalized, and learner-centric learning environment. To support this emerging approach, modern instructional design approaches and technologies must be successfully integrated into existing schoolhouse curricula.
One such approach is self-regulated learning (SRL). SRL is a process that trains learners to form skills (Zimmerman et al., 2002) and habits (Butler, 2002) that support the act of learning such as goal setting, self-monitoring, and adapting to performance (Harris & Graham, 1999; Schunk, 1996). The development of SRL abilities can lead to not just better achievement but also improved self-efficacy (Zimmerman et al., 2002). Self-efficacy is an individual’s belief in his or her ability to perform actions needed to reach goals in a specific area (Bandura, 1986).
This article investigates the effectiveness of implementing learning tools to support SRL in a military classroom context. Specifically, the extent to which learning technology can support SRL and soldier self-efficacy and impact course performance was evaluated. To address this topic, the PERvasive Learning System (PERLS) was utilized. PERLS is a mobile-first learning technology platform designed specifically to incorporate modern instructional design approaches including mobile microlearning, SRL, and adaptive learning. Developed by the Advanced Distributed Learning (ADL) Initiative, PERLS was developed in part to support the U.S. military’s broad efforts to modernize training through new technology platforms.
Microlearning
Microlearning is a learning approach based on small learning units and short‐term, focused activities (Hug et al., 2006; Lindner, 2007). They are normally less than five minutes in length (Jahnke et al., 2020). This short but integrated method of learning has been increasing in popularity over recent years in both publication trends and internet searches (Leong et al., 2020) and has grown within the training industry (Taylor & Hung, 2022). There is evidence within the literature that microlearning can support retention of information (Taylor & Hung, 2022) and build confidence of students (Hesse et al., 2019; Pascual et al., 2018). The main recommended method of implementation is mobile. Presenting microlearning content on a personal smartphone or tablet takes advantage of opportunities for engagement outside the classroom, which tend to be shorter than traditional courses. In addition, using mobile devices facilitates access to information at the point of need (Craig & Schroeder, 2020). Mobile microlearning has also been shown to improve student participation, achievement, and learning (Nikou & Economides, 2018; Suartama et al., 2019).
Self-Regulated Learning
The self-regulated learning theory decomposes learning processes into recursive phases that are enacted strategically and intentionally to improve performance (Alexander et al., 1998; Panadero, 2017; Winne, 2011; Winne & Hadwin, 2008). A task definition phase describes students’ efforts to understand the pertinent problems and available resources. The general SRL cycle is to first engage in a goal setting and planning phase to establish objectives and select tools and strategies to meet those objectives. Next, an enactment or engagement phase describes how students implement and choose strategies as well as attempt to perform the task. Finally, in an evaluation or adaptation phase, students assess their actions and outcomes and make efforts to revise their goals, plans, and strategies.
In contrast with SRL-guided learning, when students are unguided (i.e., receive minimal strategy instruction or supporting scaffolds) within a system, they are typically poor at regulating their own learning (Winne, 2005); they overestimate their abilities (Kruger & Dunning, 1999) and content understanding (Glenberg et al., 1982). As a result, students without strong SRL strategies need additional scaffolds to guide them through the process. Without guidance, the student may flounder (Kirschner et al., 2006).
Existing studies have considered the role and assessment of metacognitive monitoring and regulation in learning from multimedia, hypermedia, and educational technology (e.g., Azevedo et al., 2010). Such studies consistently link self-regulatory strategies to improved learning and performance when studying in distributed multimedia environments. Moreover, these studies demonstrate how self-regulation strategies can be taught or encouraged through various scaffolds (e.g., Azevedo & Cromley, 2004) and have demonstrated interactions between self-regulation and cognitive factors (e.g., prior knowledge; Taub et al., 2014) and motivational factors (e.g., achievement goals; Duffy & Azevedo, 2015).
PERLS and PERLS Development
The PERLS platform is the culmination of several years of research and development aimed at addressing questions about the effectiveness of technology designed to support SRL (Craig, Barnard, et al., 2022; Freed et al., 2017; Udell, 2019, 2022). While research suggests SRL improves learning outcomes, it is not a process that learners often utilize on their own (Panadero et al., 2017). Consequently, tools that facilitate engagement in SRL are beneficial to its effectiveness (Azevedo, 2005; Barber et al., 2011; Persico & Steffens, 2017). Learners must learn how to execute SRL successfully and tools to enable that process are critical to its success.
PERLS is a government-owned, off-the-shelf product that uses advanced algorithms to provide tailored learning recommendations to learners based on their characteristics, learning history, training requirements, and context. PERLS was designed primarily for mobile learning use but also provides content through a web interface. PERLS has advanced from a research and development prototype on iOS without an authoring system (Freed et al., 2017) to a robust multifunction platform system capable of creating and distributing content, supporting learning and training organizations across the Department of Defense learning ecosystem (Craig, Barnard, et al., 2022; Udell, 2019, 2022). The system has been independently user-tested with both formative expert evaluations and summative user-based testing to ensure the system works as intended and is ready for transition into learning ecosystems. This has resulted in a learning technology system that is mobile, content-agnostic, stable and scalable, empirically validated, technically documented, and designed for transition to sustainment (Craig, Siegle, et al., 2022).
Instructional Methodologies in PERLS
PERLS was specifically designed to support modern instructional design approaches. What makes PERLS unique as a learning platform is the deliberate integration of features and functions that facilitate SRL, mobile microlearning, and adaptive learning into one application. For PERLS, a learning-science-based approach drove the design of many of the functions of the software itself. Examples of this include extensive features that can support SRL (Roscoe & Craig, 2022). The system supports the planning phase of SRL through goal setting and topic selection. Planning is also enabled through search-and-discover (drill-down topics) features and a recommendation engine to support content identification. Enacting is supported by PERLS content cards (e.g., article cards, flip cards, and tip cards; see Figure 2 in Methods). The system supports reflecting exercises with the recommendation system using quiz cards and flip cards after content has been learned for reinforcement. The authoring tools in PERLS drive the content developer to create short-form content suitable for consumption on a mobile device. Instead of long-form articles, authors are supported in developing content cards to highlight key content elements, multimedia content such as videos, and learning support features such as quizzes and flashcards. These microlearning techniques allow for the learner to use PERLS for short periods of time, to supplement ongoing classroom work, and to find useful information at the point of need that can improve performance (Craig & Schroeder, 2020; Jahnke et al., 2020).
Current Study
The current study evaluates if PERLS can impact SRL, self-efficacy, and later behavior within an Army schoolhouse setting with active soldiers. Since the system is providing learning materials that support both microlearning principles and SRL, which have been shown to increase both perceptions of learning and increase learning, it is possible to make four hypotheses and testable predictions.
(H1) Use of PERLS would increase self-efficacy for materials being learned. In the current study, this would predict that soldiers who use PERLS would have higher self-efficacy for completing air assault tasks than soldiers in the study who did not use PERLS.
(H2) Because the system supports users in performing SRL behaviors, use of PERLS would increase self-efficacy for their ability to implement SRL. This would predict that soldiers who use PERLS would have higher self-efficacy for their ability to implement SRL strategies than soldiers in the study who did not use PERLS.
(h2) Because the system supports users in performing SRL behaviors, use of PERLS would increase the likelihood of implementing SRL behaviors. This would predict that soldiers who use PERLS would have report greater SRL behaviors than soldiers in the study who did not use PERLS.
(H4) Finally, the use of PERLS should increase performance within the learning setting that it is implemented. It is predicted that soldiers using PERLS would have a better completion rate due to better performance than soldiers who did not use PERLS.
Methods
Design and Setting
A randomized control trial was implemented to evaluate the usage and impact of PERLS within a military classroom setting. Participants were recruited among soldiers taking four classes at The Sabalauski Air Assault School (TSAAS). The TSAAS is an Army professional military education and training facility located in Fort Campbell, Kentucky. The schoolhouse prepares students for air insertion and air assault missions utilizing a fast-paced, and physically demanding 10-day course schedule. Students are given highly technical training from subject matter experts in both classroom and hands-on formats and tested under high-stress conditions. Due to the large amount and challenging nature of the subject material, and the requirement to rapidly apply learned material in a high-stress testing situation, air assault schools have notoriously high failure rates. However, if the student performs all required tasks to standard, she or he graduates and is certified to perform air assault tasks upon return to the operational unit.
The Air Assault Course consists of three phases of roughly three days each. Phase 1 introduces soldiers to a wide variety of basic air assault topics (e.g., facts about rotary-wing aircraft, medical evacuation, hand-arm signaling). Phase 2 involves learning how to inspect the rigging of cargo attached to rotary-wing aircraft for errors that would endanger in-flight operations. Phase 3 teaches soldiers how to rappel out of rotary-wing aircraft. At each phase, soldiers must pass both a 50-item multiple-choice test and hands-on test activities to proceed to the next phase of the course. Discussions with Air Assault Course instructors and staff identified three areas to be targeted in the project: (1) Phase 1 lecture content, (2) Phase 2 lecture content, and (3) Phase 2 hands-on training. A performance of 70% correct on a multiple-choice class assessment was necessary to pass each phase. Additionally, passing the Phase 2 hands-on test involved identifying three of four rigging errors on each of four different types of cargo loads in under two minutes each. If soldiers failed any of their tests, they were given an additional chance to pass. Notably, of the four cargo loads that soldier must learn to inspect, one of them was the most challenging and accounted for most of the Phase 2 failures (the A22 Cargo Bag). We included content in PERLS that targeted A22 Cargo Bag inspection specifically and did not include information on the other loads.
At the start of each class, the research team recruited learners in the class, collected their consent, identified pretest data, and applied the preset randomization scheme to place participants into conditions (Control or PERLS). Soldiers in the Control condition received their TSAAS course as normal with some additional material on resilience and an overview of self-regulation. Soldiers assigned to the PERLS condition were given a link and instructions on how to access PERLS in addition to receiving their standard TSAAS course. All participants were contacted via email at the end of Phase 1 and Phase 2 to complete posttest measures. The data from this article is part of the data collected from the larger study.
Participants
This study recruited 441 soldiers from four classes. A total of 16 participants were removed from analysis; five due to retaking the course or being assigned to another and 11 for dropping out of the class before any data was collected. This resulted in 425 soldiers participating in the final study. These soldiers were randomly assigned to either the PERLS condition (standard classroom with PERLS support) or the Control condition (classroom + SRL and resilience training). This resulted in 215 soldiers assigned to PERLS (PERLS condition) and 210 soldiers assigned to classroom comparison condition (Control condition). However, there were treatment adherence issues in the study with soldiers not complying with their assigned conditions. Thirty-four soldiers assigned to the Control condition signed up for PERLS accounts (it should be noted that only eighteen of the 34 used PERLS), which resulted in 192 remaining from the original assignment. Additionally, of the 215 soldiers assigned to the PERLS condition, only 87 used PERLS during the study with 128 never opening PERLS. Because of this treatment adherence effect in the data, conditions based on treatment dosage were deemed more appropriate for answering the research questions. This resulted in 320 (192+128) soldiers who did not use PERLS (New Control condition) and 105 (87+18) soldiers that used PERLS (New PERLS condition).
Independent t-test results confirmed that the PERLS and Control group had similar demographics. There were no statistically significant differences between the groups in rank, education, or time in service. Further, there were no differences in participants’ perceptions of the usefulness or familiarity with traditional formal instructional methods or informal instructional methods. The researchers concluded that these randomly assigned groups were comparable.
Additional treatment adherence problems occurred during posttest with many participants not completing the out-of-class posttest measures. Only 25 participants completed both pretest and posttest measures to be included in these analyses.
Materials/Content
PERLS Condition. For the PERLS condition, participants interacted with PERLS as an add-on to their air assault training. The PERLS content covered the material from Phase 1 and 2 of the course. The content was created by the ASU team using TSAAS class PowerPoint charts, instructor guidance packets for each topic, and the course handbook. All content was vetted by ADL Initiative instructional designers and TSAAS instructors from Fort Campbell.
Content Design. The content was created to use all aspects of PERLS, including article cards, flashcards, tip cards, two 100-item self-assessment tests, and the TSAAS handbook divided up into subsections (see Figure 2 for examples). All content in PERLS was created based on TSAAS content that was also available to soldiers taking the class. So, this condition was informationally equivalent to the Control condition. PERLS content was created following best practices based on science of learning recommendations (Craig & Schroeder, 2020). Examples of these include using deep-level multiple-choice questions with immediate feedback, reinforcement learning with flashcards, as well as articles that have visual organizers and links to provide contiguity for learning and short amounts of bite-sized information (Craig et al., 2020; Jahnke et al., 2020).
Control Condition. For the Control condition, participants took their class as normal. They were also provided additional training material on resilience and self-regulated learning. This content was identical to the content provided to students within the PERLS condition. However, it was provided as a supplemental online document. All interaction within this condition was between the participant and human instructors.
Self-Efficacy Assessment. A modified version of the General Self-Efficacy Scale (Chen et al., 2001) was used for this study at pre- and posttest to determine soldiers’ self-efficacy. This test has eight items and is measured on a five-point scale (Strongly Disagree, Disagree, Slightly Agree, Agree, and Strongly Agree). One version of the scale was modified to assess soldiers’ self-efficacy regarding their self-regulation abilities. A second version of the scale was modified to assess soldiers’ self-efficacy with respect to their ability to perform air assault tasks (for the modified scale, see Craig, Siegle et al., 2022, Appendix). Both measures were given at pretest and posttest.
Self-Regulated Learning Measure. A version of the Kocdar et al. (2018) Self-Regulated Learning Measure was implemented to detect learners’ self-regulation behaviors at pretest and posttest. This assessment has a total of 30 questions across five subscales: Goal Setting (5), Help Seeking (9), Self-Study Strategies (8), Managing Physical Environment (6), and Effort Regulation (2). This measure uses a five-point scale: Strongly Disagree, Disagree, Slightly Agree, Agree, and Strongly Agree.
Soldier Course Completion. Course completion was defined as a participant starting the class, consenting to participate in the study, and completing the course at the end of Phase 2 as indicated by the schoolhouse.
Procedure
Soldiers were recruited using a short in-person presentation within classrooms. Each soldier was given a research packet that included a consent form, initial instructions, a pretest knowledge measure, pretest version of the self-efficacy scales, pretest version of the SRL scale, and an instruction page on next steps depending on their condition. Soldiers in the PERLS conditions were given instructions on downloading and creating an account in PERLS. Soldiers in the Control condition received a link to two PowerPoint files on self-regulated learning and resiliency. It was up to participants to follow links and instructions provided. The day before the final test in Phase 1, all participants were contacted via email with a link to Phase 1 self-efficacy assessments. The participating soldier was contacted again via email the day before the Phase 2 assessment.
Results
Self-Efficacy for Air Assault Tasks
An analysis of covariance (ANCOVA) was conducted on soldiers’ post training self-efficacy for air assault task ability using pretest self-efficacy measures for air assault tasks as covariates to determine any difference between condition and classes. This test indicated a significant difference for PERLS usage, F(1, 16) = 5.48, p = .03; ηp2 = .26, but not for class or the interaction. Soldiers that interacted with PERLS (M = 4.39, SD = .62) had significantly higher self-efficacy than soldiers that did not interact with PERLS (M = 3.70, SD = .45) (see Table 1).
Self-Efficacy for Self-Regulated Learning
An ANCOVA was conducted on soldiers’ posttraining self-efficacy for SRL ability using pretest self-efficacy measures for SRL as covariates to determine any difference between conditions. This test indicated a significant difference between PERLS and Control groups, F(1, 16) = 6.16, p = .02; ηp2 = .28. Soldiers that interacted with PERLS (M = 4.13, SD = .63) had significantly higher self-efficacy than soldiers that did not interact with PERLS (M = 3.62, SD = .55) (see Table 2).
Self-Reported SRL Behaviors
A series of ANCOVAs were conducted on participants’ reported self-regulated learning ability and on the five subscales for the SRL measure using corresponding pretest measures as covariates to determine any difference between PERLS usage conditions and classes. This test did not indicate any significant differences. The pre- to post-measures were generally in favor of slight improvement for the PERLS condition. While the effect size was generally small to medium, it is possible that the nonsignificant effect is due to small sample size. Additionally, it should be noted that these means and the results could be biased due to the low sample size and attrition.
Student Retention
A one-tailed t-test was conducted on soldiers’ completion rates to determine differences between PERLS usage conditions. The variances between groups were not equal, so a corrected model was used to interpret the data. This test indicated a significant difference, t(224) = 5.08, p = .001, d = 0.51. Soldiers who interacted with PERLS (M = .18, SD = .39) had significantly more retention than the Control condition (M = .42, SD = .49). The means roughly indicate the proportion of soldiers who did not finish the course within the specific condition. This analysis shows that participants that used PERLS dropped the course at half the rate of participants that did not use PERLS.
Discussion
Overall, the combination of a mobile microlearning system with support for SRL processes as seen within PERLS had a positive impact on soldiers’ perceptions and completion rates and generally supported our hypotheses. Our first hypothesis (H1) that use of PERLS would support soldiers’ self-efficacy for air assault tasks was supported. Our second and third hypotheses regarding the use of PERLS supporting soldiers SRL received mixed results with reported self-efficacy for SRL showing increases (H2) but reported SRL behavior not showing increased (h2) compared to controls. Our final hypothesis on classroom impact (H4) was also supported with increased completion rates for soldier using PERLS.
The discrepancy in SRL findings could be explained by the short duration of the implementation. Self-efficacy is a person’s belief in their ability to perform a behavior or set of behaviors (Bandura, 1977, 1986, 1997). Self-efficacy has been shown to directly impact motivation that leads to skill transfer (Chiaburu & Marinova, 2005). Elevated self-efficacy increases the intent to perform the learned skills so that the next step of doing is initiated (Machin & Fogarty, 1997). However, the sensitivity of the self-regulation behaviors measure used in the current study may have been impacted by the limited scope of the evaluation. The measure was designed to assess long-term changes in learning behavior, while our study was constrained to the context of one course. So, it is possible that the scale was not sensitive enough to capture fine grained changes from within the course. Future research investigating the longer lasting impacts of the intervention on SRL behavior would be required to determine the extent to which substantial changes to SRL behaviors would result from using PERLS.
Improving Classroom Completion Rates with PERLS
The study shows that soldiers using PERLS completed the course at significantly higher rates than those that did not. In interpreting this finding, consideration is needed of the treatment adherence effect in that many participants assigned to use PERLS did not use it due to unknown reasons. This was perhaps due to the short duration of the course with a high amount of optional content and PERLS as part of the class. While pretest data indicate that the treatment adherence problem was random without any statistical differences between Control, PERLS, and the original groups with the treatment adherence problems, it is always possible that there was another systematic factor that we could not identify. However, this does not seem highly plausible due to the similar pretest findings. Additionally, the current findings align with the previous research on learning technologies (Foster & Fletcher, 2002), microlearning systems (Jahnke et al., 2020; Nikou & Economides, 2018), SRL support systems (Azevedo et al., 2010), and other findings with the current PERLS (Craig, Siegle, et al., 2022), all of which have consistently been shown to increase learning. However, the use rate within the PERLS condition of 40% highlights the need to better understand soldier hesitancy in adoption of learning technology and what might increase use rates of learning technology.
Our evaluation found that PERLS was an effective system that was generally viewed as helpful by users and instructors who would recommend reuse within the course. This finding also aligns well with some of our qualitative findings (Craig, Barnard, et al., 2022). Soldier interviews pointed toward the successful use of PERLS during the class. Interviews with instructors did not report any negative impacts, increased disruption, or increased instructor burden from the implementation of PERLS.
While the increase in completion rates seems promising, the return on investment should be examined for courses before adoption, as the manpower required to develop and maintain course content within PERLS is significant. The Cadre of TSAAS were not asked to create the materials used in this evaluation, so the ability for these instructors to create content within PERLS is unknown and additional attention and research should focus on the interface and usability of the system for content creation and maintenance.
PERLS’ Broader Potential Impact
The U.S. Army Learning Concept (U.S. Department of the Army, 2017) recognizes a need for mobile microlearning as part of a larger learning ecosystem, with the ability to adapt to shifting educational demands of the individual. The current study has shown positive evidence for the adoption of PERLS into military education and training environments. The convenience of having on-demand training materials available in an online application allows soldiers to study wherever and whenever they can fit it into their busy training schedules. PERLS provides immediate feedback to the learner about their current knowledge on a subject, providing a tailored approach to learning soldiers are unable to receive through individual handbook study alone. Web or application-based microlearning solutions, like PERLS, may provide postgraduation access to the software for soldiers to review important materials after they return to their rotational units, allowing them reach back capability, increase their knowledge retention duration, and remain subject-matter experts in applied operational environments.
Conclusions
The current study has shown positive evidence for the adoption of PERLS into military education and training environments. It found that PERLS use during a course could (1) improve soldiers’ self-efficacy for both content and SRL and (2) potentially increase retention/completion rates for soldiers. SRL has notoriously been difficult to instill without intense training (Winne, 2005), and these findings show the possibility that correctly designed technology can support user’s self-efficacy for their ability to implement SRL without explicit instruction. Further, this study has shown that technology that combined SRL support with a microlearning platform, as seen in PERLS, has the potential for real-world impact within classrooms.
Acknowledgements
The current article was funded by HQ0034-19-C-0018, a contract from the United States Department of Defense Advanced Distributed Learning Initiative. The statements within this work do not reflect the opinion, views, or policy of the funding agency.
References
Alexander, P. A., Graham, S., & Harris, K. R. (1998). A perspective on strategy research: Progress and prospects. Educational Psychology Review, 10(2), 129–154. https://doi.org/10.1023/A:1022185502996
Azevedo, R. (2005). Using hypermedia as a metacognitive tool for enhancing student learning? The role of self-regulated learning. Educational Psychologist, 40(4), 199–209. https://doi.org/10.1207/s15326985ep4004_2
Azevedo, R., & Cromley, J. G. (2004). Does training on self-regulated learning facilitate students’ learning with hypermedia? Journal of Educational Psychology, 96(3), 523–535. https://doi.org/10.1037/0022-0663.96.3.523
Azevedo, R., Johnson, A., Chauncey, A., & Burkett, C. (2010). Self-regulated learning with MetaTutor: Advancing the science of learning with MetaCognitive tools. In M. S. Khine & I. M. Saleh (Eds.), New science of learning: Cognition, computers and collaboration in education (pp. 225–247). Springer.
Bandura, A. (1977). Self-efficacy: Toward a unifying theory of behavioral change. Psychological Review, 84(2), 191–215. https://doi.org/10.1037/0033-295X.84.2.191
Bandura, A. (1986). Social foundations of thought and action: A social cognitive theory. Prentice-Hall.
Bandura, A. (1997). Self-efficacy: The exercise of control. W. H. Freeman.
Barber, L. K., Bagsby, P. G., Grawitch, M. J., & Buerck, J. P. (2011). Facilitating self-regulated learning with technology: Evidence for student motivation and exam improvement. Teaching of Psychology, 38(4), 303–308.
Butler, D. (2002). Individualizing instruction in self-regulated learning. Theory into Practice, 41(2), 81–92. https://doi.org/10.1207/s15430421tip4102_4
Chen, G., Gully, S. M., & Eden, D. (2001). Validation of a new general self-efficacy scale. Organizational research methods, 4(1), 62–83.
Chiaburu, D.-S., & Marinova, S. V. (2005). What predicts skill transfer? An exploratory study of goal orientation, training self‐efficacy and organizational supports. International Journal of Training and Development, 9(2), 110–123. https://doi.org/10.1111/j.1468-2419.2005.00225.x
Craig, S. D., & Schroeder, N. L. (2020). Science of learning and readiness (SoLaR) recommendation report: Science of learning practices for distributed online environments. Arizona State University.
Craig, S. D., Barnard, W. M., Gordon, K. E., Riddle, D. L., & Milham, L. M. (2022). The impact of the PERvasive learning system (PERLS) on stakeholders’ perceptions and classroom retention. In Proceedings of Interservice/Industry Training, Simulation and Education Conference (Paper 22468). National Training & Simulation Association.
Craig, S. D., Siegle, R. F., Li, S., Cooper, N. R., Liu, Y., & Roscoe, R. D. (2022). An investigation of the PERvasive learning systems impact on soldiers’ self-efficacy for self-regulation skills. In Proceedings of the Human Factors and Ergonomics Society Annual Meeting (pp. 742–746). Sage.
Duffy, M. C., & Azevedo, R. (2015). Motivation matters: Interactions between achievement goals and agent scaffolding for self-regulated learning within an intelligent tutoring system. Computers in Human Behavior, 52, 338–348. https://doi.org/10.1016/j.chb.2015.05.041
Foster, R. E., & Fletcher, J. D. (2002). Computer-based aids for learning, job performance, and decision-making in military applications: Emergent technology and challenges. Institute for Defense Analyses. https://apps.dtic.mil/sti/citations/ADA413872
Freed, M., Yarnall, L., Spaulding, A., & Gervasio, M. (2017). A mobile strategy for self-directed learning in the workplace. In Proceedings of the Interservice/Industry Training, Simulation and Education Conference (Paper 17265). National Training & Simulation Association.
Glenberg, A. M., Wilkinson, A. C., & Epstein, W. (1982). The illusion of knowing: Failure in the self-assessment of comprehension. Memory & Cognition, 10(6), 597–602. https://doi.org/10.3758/BF03202442
Harris, K., & Graham, S. (1999). Programmatic intervention research: Illustrations from the evolution of self-regulated strategy development. Learning Disability Quarterly, 22(4), 251–262. https://doi.org/10.2307/1511259
Hesse, A., Ospina, P., Wieland, M., Yepes, F. A. L., Nguyen, B., & Heuwieser, W. (2019). Short communication: Microlearning courses are effective at increasing the feelings of confidence and accuracy in the work of dairy personnel. Journal of Dairy Science, 102(10), 9505–9511. https://doi.org/10.3168/jds2018-15927
Hug, T., Lindner, M., & Bruck, P. A. (Eds.). (2006). Microlearning: Emerging concepts, practices and technologies after e-learning. Innsbruck University Press.
Jahnke, I., Lee, Y. M., Pham, M., He, H., & Austin, L. (2020). Unpacking the inherent design principles of mobile microlearning. Technology, Knowledge and Learning, 25(3), 585–619. https://doi.org/10.1007/s10758-019-09413-w
Kirschner, P. A., Sweller, J., & Clark, R. E. (2006). Why minimal guidance during instruction does not work: An analysis of the failure of constructivist, discovery, problem-based, experiential, and inquiry-based teaching. Educational Psychologist, 41(2), 75–86. https://doi.org/10.1207/s15326985ep4102_1
Kocdar, S., Karadeniz, A., Bozkurt, A., & Buyuk, K. (2018). Measuring self-regulation in self-paced open and distance learning environments. International Review of Research in Open and Distributed Learning, 19(1), 25–43. https://doi.org/10.19173/irrodl.v19i1.3255
Kruger, J., & Dunning, D. (1999). Unskilled and unaware of it: How difficulties in recognizing one’s own incompetence lead to inflated self-assessments. Journal of Personality & Social Psychology, 77, 1121–1134. http://dx.doi.org/10.1037//0022-3514.77.6.1121
Leong, K., Sung, A., Au, D., & Blanchard, C. (2020). A review of the trend of microlearning. Journal of Work-Applied Management, 13(1), 88–102. https://doi.org/10.1108/JWAM-10-2020-0044
Lindner, M. (2007). What is microlearning? (Introductory Note). In 3rd International Microlearning 2007 Conference. Innsbruck University Press.
Machin, M. A., & Fogarty, G. J. (1997). The effects of self‐efficacy, motivation to transfer, and situational constraints on transfer intentions and transfer of training. Performance Improvement Quarterly, 10(2), 98–115. https://doi.org/10.1111/j.1937-8327.1997.tb00051.x
Nikou, S. A., & Economides, A. A. (2018). Mobile‐based micro‐learning and assessment: Impact on learning performance and motivation of high school students. Journal of Computer Assisted Learning, 34(3), 269–278. https://doi.org/10.1111/jcal.12240
Panadero, E. (2017). A review of self-regulated learning: Six models and four directions for research. Frontiers in Psychology, 8, Article 422. https://doi.org/10.3389/fpsyg.2017.00422
Pascual, K. J., Vlasova, E., Lockett, K., Richardson, J., & Yochelson, M. (2018). Evaluating the impact of personalized stroke management toolkits on patient experience and stroke recovery. Journal of Patient Experience, 5(4), 244–249. https://doi.org/10.1177/2374373517750416
Persico, D., & Steffens, K. (2017). Self-regulated learning in technology enhanced learning environments. In R. Carneiro, P. Lefrere, K. Steffens, & J. Underwood (Eds.), Technology enhanced learning (pp. 115–126). Springer.
Roscoe, R. D. & Craig, S. D. (2022). A heuristic assessment framework for the design of self-regulated learning technologies. Journal of Formative Design in Learning, 6, 77–94. https://doi.org/10.1007/s41686-022-00070-4
Schunk, D. (1996). Goal and self-evaluative influences during children’s cognitive skill learning. American Educational Research Journal, 33, 359–382. https://doi.org/10.3102/00028312033002359
Suartama, I. K., Setyosari, P., Sulthoni, S., & Ulfa, S. (2019). Development of an instructional design model for mobile blended learning in higher education. International Journal of Emerging Technologies in Learning, 14(16), 4–22. https://doi.org/10.3991/ijet.v14i16.10633
Taub, M., Azevedo, R., Bouchet, F., & Khosravifar, B. (2014). Can the use of cognitive and metacognitive self-regulated learning strategies be predicted by learners’ levels of prior knowledge in hypermedia-learning environments? Computers in Human Behavior, 39, 356–367. https://doi.org/10.1016/j.chb.2014.07.018
Taylor, A. D., & Hung, W. (2022). The effects of microlearning: A scoping review. Educational Technology Research and Development, 70, 1–33. https://doi.org/10.1007/s11423-022-10084-1
Udell, C. E. (2019, August). PERLS V2.0 progressing from research to production [Poster presentation]. iFEST 2019, Alexandria, VA, United States. https://www.ntsa.org/events/2019/8/26/91d0/poster-gallery/ifest-2019-poster-gallery
Udell, C. E. (2022, August). Self-regulated learning and adaptive content delivery [Poster presentation]. iFEST 2022, Alexandria, VA, United States. https://www.ntsa.org/events/2022/8/16/ifest-2022/poster-gallery/ifest-2022-poster-gallery
U.S. Department of the Army. (2017). The U.S. Army Learning Concept for Training and Education (TRADOC Pamphlet 525-8-2). U.S. Government Publishing Office.
Winne, P. H. (2005). A perspective on state-of-the-art research on self-regulated learning. Instructional Science, 33(5), 559–565. https://doi.org/10.1007/s11251-005-1280-9
Winne, P. H. (2011). A cognitive and metacognitive analysis of self-regulated learning. In D. H. Schunk & B. Zimmerman (Eds.), Handbook of self-regulation of learning and performance (pp. 15–32). Routledge.
Winne, P., & Hadwin, A. (2008). The weave of motivation and self-regulated learning. In D. H. Schunk & B. Zimmerman (Eds.), Motivation and self-regulated learning: Theory, research, and applications (pp. 297–314). Lawrence Erlbaum Associates.
Zimmerman, B., Bonner, S., & Kovach, R. (2002). Developing self-regulated learners: Beyond achievement to self-efficacy. American Psychological Association.
Scotty D. Craig is an associate professor of human systems engineering within the Ira A. Fulton Schools of Engineering at Arizona State University (ASU) and an affiliate faculty of the Mary Lou Fulton Teachers College and the director of the ASU Advanced Distributed Learning Partnership Laboratory. He is a learning engineer working at the intersection of cognitive science, science of learning, and user sciences to design and evaluate learning systems.
Dawn L. Riddle is a SETA contractor for the Advanced Distributed Learning Initiative, serving as test and evaluation manager and learning team lead. She earned a PhD in industrial and organizational psychology from the University of South Florida and has experience across academia, industry, and government specializing on the design, development, and evaluation of learning and education and training solutions for complex work environments.
Dr. Shanda Lauer is a research psychologist, working in the Institutional Research and Assessment Division, Vice Provost of Academic Affairs, at the Army University in Fort Leavenworth, Kansas. She holds a master’s degree in biology focusing in discipline-based education research, a certification in college science teaching, and a PhD in psychology with a neuroscience emphasis. Over the past four years, she has managed the Army University Research program, and coordinated the Learning Sciences Committee, both of which seek to promulgate emerging research within the learning sciences throughout the Army Learning Enterprise. Her own program of research focuses on improving communication in the Army and enhancing education through technology use and the application of best practices.
Gregory Hughes is a research psychologist at the U.S. Army Combat Capabilities Development Soldier Center at Natick, Massachusetts. Hughes obtained a PhD in experimental psychology from Tufts University and has been conducting Army research for six years. His main research efforts focus on optimizing the acquisition and retention of new knowledge and complex skills. As part of this work, he has conducted research on using mobile applications, virtual-reality simulations, and in-person training techniques to enhance outcomes in Army Schoolhouse settings. Complementing this work, he also investigates methods of mitigating the impact of acute stress on learning and memory.
Wade R. Elmore received his PhD in psychology from the University of Missouri–Kansas City in 2014. Elmore began working with the Army Research Institute for Behavioral and Social Sciences as a student research fellow in 2011 and as a postdoctoral research fellow where he found a passion for civilian service in support of the soldiers of the U.S. Army. In his ten years working for the U.S. Army, Elmore has worked at the Center for Army Leadership, The Army University, and in 2021 joined the Cognitive Sciences and Applications Team of Combat Capability Development Command–Soldier Center. Elmore has contributed to the enterprise level understanding of Army leadership and Army professional military education using Army-wide surveys. Currently, Elmore is engaged in research examining the use of learning sciences best practices on military education and training, and the efficacy of applying these best practices in classroom instruction and through distributed asynchronous training and education platform, characterizing soldier-relevant cognitive and physical traits, and characterizing tactical performance during sustained live-fire exercises.
Chad E. Udell is chief strategy officer and cofounder of Float, an application development firm that designs and builds tools for learning and performance. As a three-time published author on adult-learning technology and related topics, Udell is focused on user-experience and user-interface design that creates frictionless learning experiences for his customer’s audiences. Udell brings over 20 years of experience building digital products to his collaborative efforts with partners and clients.
Laura M. Milham is an Air Force civilian and an applied human-systems scientist, with an emphasis on individual and team training and assessment. She earned a PhD from the University of Central Florida. As the acting Advanced Distributed Learning director, Milham brings over 25 years of experience to oversee the program’s comprehensive execution, guiding its strategy, and ensuring it aligns with U.S. Department of Defense and federal government priorities.
Back to Top