Air Assault!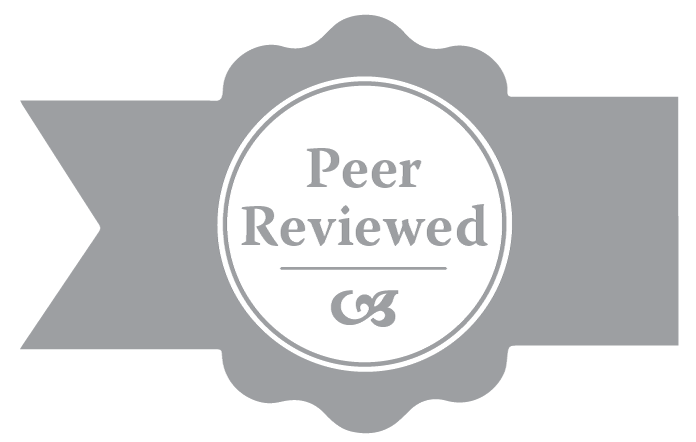
Applying Learning Science to Army Skill and Knowledge Acquisition
Gregory I. Hughes1,3, Shanda D. Lauer2, and Wade R. Elmore1
1 U.S. Army Combat Capabilities Development Command Soldier Center
2 Institutional Research and Assessment Division, Vice Provost of Academic Affairs, Army University
3 Center for Applied Brain and Cognitive Sciences
Download the PDF 
To ensure force readiness, soldiers in the U.S. Army must acquire critical knowledge and skills at an incredible rate. They are expected to retain and recall this knowledge throughout their careers not only in garrison environments but also in austere, high-stakes, and stressful conditions. As time and resources available for training and education are constrained, it is imperative to optimize these activities using all the resources available to the Army. Although Army schools are highly successful at preparing soldiers for their duties, there are techniques that could improve education and training that have been underexplored in military contexts. Over the past several decades, researchers in the cognitive sciences have identified techniques that reliably enhance long-term learning outcomes, even with little to no investment of time or resources (for relevant reviews, see Cepeda et al., 2006; Firth et al., 2021; Hughes & Thomas, 2021). However, these techniques have overwhelmingly been explored in laboratory settings, civilian educational environments (i.e., kindergarten to college), and sports. The purpose of this study was to explore how learning techniques that require minimal investment of time and resources could be integrated into an Army education and training environment. Specifically, we partnered with the Sabalauski Air Assault School at Fort Campbell, Kentucky, to explore these research questions.
Learning Sciences
Among the most potent learning techniques are practice testing, spacing out learning sessions, and interleaving learning materials. Research overwhelmingly demonstrates that practice testing leads to superior learning compared to an equivalent amount of time reviewing material (for a review, see Adesope et al., 2017). The superiority of practice testing has not only been documented when compared to less effortful study methods like rereading and highlighting but also to deeper, conceptual, and/or elaborative methods of studying (e.g., idea mapping, sentence generation, and creating mnemonic devices; see Karpicke & Blunt, 2011; Karpicke & Smith, 2012). Spacing is another potent technique. The spacing effect refers to the finding that it is better to spread out the studying of a topic into multiple instances across time compared to an equal amount of time studying that topic in a single session (e.g., a one-hour learning session on four separate days compared to a single four-hour learning session) (Ebbinghaus, 1885; for reviews, see Cepeda et al., 2006; Delaney et al., 2010). Relatedly, interleaving is a method of reviewing material that is similar to the spacing effect but carries an additional advantage. The interleaving effect is the finding that studying various topics in an alternating fashion (ABABABAB) is often better than studying one topic entirely before moving onto another (i.e., blocking: AAAABBBB; e.g., Goode & Magill, 1986; Hall et al., 1994; Kornell & Bjork, 2008; for a review, see Firth et al., 2021). Interleaving necessarily involves some degree of spaced learning, since the study of one topic is divided into temporally distinct instances. A unique benefit of interleaving is that it juxtaposes different topics, allowing learners to compare and contrast the shared and distinct features of each topic. This juxtaposition, termed discriminative contrast, is useful when categories of knowledge share many features in common, making it difficult for learners to notice the subtle differences that separate them (Goldstone, 1996; Kornell & Bjork, 2008; for a review, see Hughes & Thomas, 2021).
Although these learning techniques entail their own unique advantages, their efficacy is underpinned by similar mechanisms. There are two mechanistic frameworks that parsimoniously explain these benefits. One is the principle of transfer-appropriate processing (Blaxton, 1989; Morris et al., 1977), which states that performance is optimized when the cognitive processes involved in training match those that are called upon during the later testing of those skills. This framework explains why practice testing is effective, as it requires people to recall information from long-term memory, which is precisely what is normally asked of them during their graded exams. Similarly, spacing is effective because when learners are assessed, there has usually been an appreciable amount of time since the last study episode. Spaced learning approximates the experience they will later have when their knowledge or skill level is formally assessed. Another is the principle of desirable difficulty (Bjork, 1994; Bjork & Bjork, 2020), which states that learning is optimized when people are practicing at a moderate level of difficulty. The most used learning techniques are shallow and low effort (e.g., rereading), keeping the level of challenge too low to spur sufficient growth and progress.
To determine where and how these techniques could be implemented at the Sabalauski Air Assault School at Fort Campbell, Kentucky, we conducted focus groups and interviews with the instructor cadre. Overwhelmingly, the cadre expressed that a single component of the air assault course resulted in more failures than any other: identifying errors in equipment rigged to aircraft that would endanger in-flight operations (sling load inspection). In this context, the sling is the name for the equipment that attaches cargo (a load) to a rotary-wing aircraft. Incorrectly rigging the load to the aircraft can endanger in-flight operations by creating aerodynamic instability. Correct rigging is therefore vital to successful air assault operations. In the present study, we worked with the cadre to modify the training of sling load inspection and compared course outcomes with the previous methods of training.
Sling Load Inspection
In the air assault course, soldiers learn to inspect four loads (see Figure 1): the A-22 Cargo Bag, M1151 HMMWV (i.e., a humvee truck), M1102 Trailer, and 5K Cargo Net. The skill essentially consists of two simultaneous tasks: (a) performing a recommended inspection sequence, a systematic method of reviewing the equipment in a particular order/manner ensuring full coverage of the rigging and load; and (b) a categorization task in which pieces of the equipment are judged as operable or deficient (see Figure 2). The identification of deficiencies is the true focus of the task, as these are defined as errors in the rigging that would threaten the viability of safe in-flight operations.
The four types of loads. From left to right: M1151 HMMWV (humvee), A22 Cargo Bag, 5K Cargo Net, and M1102 High Mobility Trailer.
To pass the air assault course, soldiers must successfully conduct sling load inspection on four different types of loads (see Figure 1). For each load, soldiers must identify three out of four deficiencies in under two minutes. Although a specific inspection sequence is taught and strongly recommended by instructors, it is not required during testing and soldiers are not penalized for deviating from that sequence. After the first round of testing is complete, soldiers who failed any of the loads receive additional instruction and then are given a second opportunity to conduct the sling load inspection on each type of load they failed. On the second test, the sling loads may have an entirely new set of deficiencies. A soldier who fails any load twice also fails the entire course.
Note. Left: 10K Apex with no deficiency. Right: 10K Apex with a missing castellated nut in the top right corner of the equipment.
Soldiers are trained on sling load inspection through a mixture of classroom presentations, in-person lectures with the equipment, and hands-on practice (practical exercises). Learning science techniques could be integrated into any of these learning activities and/or at-home study materials. For the purposes of our project, we limited our efforts to modifications of the practical exercises that would require virtually no increase in time or resources to implement. We made this decision for three reasons. First, the majority of training time is spent on the practical exercises, meaning that an intervention in this part of the course would likely exert the largest effects on the learning outcomes. Second, modifications to the practical exercises would circumvent adherence problems that would likely occur with voluntary after-hours exercises or with at-home study materials. Third, the practical exercises are the part of the training that is most similar to the actual hands-on sling load inspection test. This means that any improvements in these exercises would be most likely to transfer to the hands-on tests.
Motivated by the principle of transfer-appropriate processing, we decided to explore how making the practical exercises more like actual testing conditions would affect course outcomes. Recall that testing conditions require soldiers to inspect loads and identify three out of four rigged deficiencies in under two minutes per load. The practical exercises deviate from these conditions in two critical ways. First, half of these exercises are performed on clean loads, which have no deficiencies rigged on the equipment, but soldiers are only presented with loads that do have deficiencies during testing conditions (dirty loads). Second, the practical exercises are not timed, meaning that soldiers never get accustomed to the feeling of time pressure and/or establish an appropriate pace and rhythm for conducting their inspections. The cadre emphasized that soldiers frequently struggled with the time pressure of their tests, causing many soldiers to go too quickly or too slowly. Therefore, we had the cadre make all the practical exercises done with (a) only dirty loads (four deficiencies rigged on the equipment) and (b) time pressure. The cadre decided to set the timers for three minutes rather than the two-minute standard used during actual testing conditions. Although this timing component did not precisely reflect testing conditions, it perhaps struck a balance between making the practical exercises more test-like and making the task too difficult for novices (i.e., two minutes may have been undesirably difficult).
Notably, conducting the practical exercises with all dirty loads challenged an intuitive notion held by many members of the cadre, which is that time spent with clean loads is uniquely valuable for honing the skill of sling load inspection. The basic idea is that by spending time with clean loads, a soldier learns “what right looks like,” and consequently, deviations from “right” would leap out at the soldier, who would then call out a deficiency. Replacing this time with more exposure to dirty loads would hypothetically put the cart before the horse, undermining the acquisition of what “right” looks like.
There is ample scientific evidence to call this notion into question. This comes from a literature on visual category learning, which investigates similar skills to sling load inspection but with different materials. Sling load inspection is fundamentally a series of discrete visual categorization tasks in which soldiers deem subcomponents of the rigging as belonging to one of two categories: functional or deficient. Although the inspection sequence involves interacting with the equipment physically, the categorization component of the task is primarily visual in nature. The deficiencies are identified based on appearances rather than tactile cues (e.g., the absence of a castellated nut, a twist in a strap, or a misrouted chain can all be identified by sight alone; see Figure 2). Visual categorization experiments, such as those that involve determining whether chest X-rays exhibit healthy lungs or signs of disease, involve the same underlying cognitive mechanisms.
In the terminology of the research on visual category learning, some members of the cadre saw value in “blocking” the study of categories (i.e., study the category of “clean” before “dirty”). Early researchers examining visual categorization felt similarly, arguing that it makes sense to master one category before moving onto another (e.g., for categories clean [C] and dirty [D], the sequence could look like: CCCCDDDD; see Gagné, 1950; Kurtz & Hovland, 1956). However, this method is usually not as effective as alternating between examples of each category (i.e., interleaving; CDCDCDCD), especially when the features that discriminate the categories are subtle deficiencies (for a review, see Hughes & Thomas, 2021), which is typical of sling load inspection (e.g., the orientation of a small castellated nut can distinguish between clean and deficient; see Figure 2). Interleaving is beneficial for learning because it highlights and draws attention to the critical differences between categories (e.g., clean vs. dirty), making the learning process more efficient by promoting discriminative contrast (Goldstone, 1996; Kang & Pashler, 2012; Kornell & Bjork, 2008). In the context of sling load inspection, interleaving would mean examining a clean version of a piece of equipment (e.g., a correctly rigged 188-inch strap) and then studying a dirty version of that equipment (a version with a deficiency; e.g., a twisted 188-inch strap). This type of juxtaposition would only occur during dirty load sessions because they entail a mixture of clean and dirty equipment. An additional benefit of this kind of study method is that it keeps learners engaged. Blocked learning sequences tend to be too predictable and result in boredom (Guzman-Munoz, 2017).
Method
Participants
We obtained data from a total of 2,826 soldiers who participated in the sling load portion of the air assault course. The treatment group consisted of six classes (N = 656). The control group was composed of the preceding fourteen classes (N = 2,170). Each class was taught by one of three instructor teams.
Procedure
The Combined Academic Institutional Review Board of Army University provided a human subjects research determination of exempt research project with concurrence from the U.S. Army Combat Capabilities Development Command Soldier Center Human Research Protections Office. The exempt categorization was due to the research occurring in normal established classroom settings, involving normal educational practices, and being unlikely to negatively impact students’ ability to learn required educational content. For the treatment classes, we had the cadre brief soldiers on our efforts to evaluate the efficacy of course modifications and inform soldiers that they could opt out of their data via a web link. No soldiers opted to withhold their data from the project.
In the treatment classes, we had the cadre modify the practical exercises in six classes by (1) replacing all clean loads (no deficiencies rigged) with dirty loads (four deficiencies rigged) and (2) introduce time pressure by limiting soldiers to three minutes per sling load practical exercise. For the control classes, we asked the cadre to provide historical data from the preceding classes, which we used as baseline performance levels. For all classes, we asked the cadre to record the performance of each soldier for each load on the initial test and the retest. We also requested the cadre provide us with individual soldier characteristics that they identified as significant predictors of performance, which included soldier rank and temporary duty status (whether a soldier was permanently stationed at Fort Campbell or was on orders from another location).
Results
We used fixed and mixed logistic regression modeling to analyze binary outcome data and adopted an alpha rate of .05. The analyses were conducted in R (R Core Team, 2022). We used the lme4 package (Bates et al., 2014) for logistic regression modeling and the emmeans package for analyzing estimated marginal means (Lenth, 2020). The primary dependent variable of interest was whether a soldier passed the hands-on sling load test. We were unable to analyze the data in a more granular way, as the schoolhouse only provided us with performance data on each test (first or retest) and each load for less than half of the collected sample (for 1,142 out of the 2,826 soldiers). An analysis on this subset of data would be problematic because we would be unable to control for several contaminating factors, the importance of which will become clear in the subsequent analysis. Note that of the six treatment classes, only four incorporated the element of time pressure. Nevertheless, we analyzed all six treatment classes as a single unit, as all of them used dirty loads during the practical exercises.
Hands-on Sling Load Test
For the hands-on sling load test, soldiers in the treatment group (M = 84.99%) outperformed those in the control group (M = 77.30%) by 7.69 percentage points, β = .51, p < .0001. However, there were differences across the groups that could have accounted for this increase in pass rate rather than the modified practical exercises. To evaluate this possibility, we examined the contribution of several variables the schoolhouse cadre identified as potential confounds, including average class size, instructor teams, and two variables pertaining to class composition (TDY status and soldier rank). Ultimately, we planned to fit a model that accounted for any of the factors that may have unfairly influenced the between-groups comparison.
Class Size. The average class of the treatment group (M = 111) was smaller than that of the control group (M = 171), suggesting the possibility that the smaller class size underlay the enhanced pass rate. However, the pass rate of the smallest 10 classes (M = 79%) was not reliably different than the largest 10 classes (M = 79%), t(18) = 0.08, p = .94, d = 0.03. We therefore did not include this variable in our final model.
Instructor Teams. The number of soldiers taught by each instructor group was not equal across groups, X2(2) = 16.69, p < .001 (see Table 1). For example, 40% of soldiers in the control group were taught by Team A, but only 31% of soldiers in the treatment group were taught by Team A. This was problematic because the overall pass rate of Team A (70%) was lower than Teams B (90%) and C (81%), suggesting a confound in the difference in pass rates among the groups.
TDY Status. Next, we looked at whether each soldier’s home station was Fort Campbell, meaning that the air assault school was local to them, or if they were traveling to attend this course from another installation (i.e., they are on temporary duty or TDY). As shown in Table 2, soldiers who were TDY (M = 88%) passed at a higher rate than those who were local (M = 77%), β = .82, p < .0001. On average, the proportion of TDY soldiers was higher in the treatment group (M = 28%) compared to the control group (M = 18%), β = .58, p < .0001, resulting in an artificial advantage of the former over the latter.
Soldier Rank. We next turned our attention to soldier rank. For the sake of a simpler analysis, we created three bins for soldier rank: junior enlisted, senior enlisted, and officer. As shown in Table 3, higher rank soldiers (M = 90%) passed at a higher rate than lower ranked soldiers (M = 76%), β = 1.10, p < .001. As shown in Table 3, the rank composition of the treatment and control groups were not identical, X2(2) = 37.13, p < .001. For example, junior-enlisted soldiers were a greater proportion of the control (M = 56%) compared to the treatment group (M = 43%). Again, this was a confound that benefited the pass rate of the treatment group.
Final Model
We used mixed-effects logistic regression to create a model that predicted the effect of treatment group on pass rates while accounting for instructor teams (random effect), TDY status (fixed effect), and rank (fixed effect). Treatment group was coded as 0 (control) or 1 (treatment); TDY status as 0 (local) or 1 (TDY); and rank as 0 (enlisted) or 1 (officer).1 We evaluated the significance of the fixed and random effects by conducting chi-square likelihood ratio tests on the change in model fit (deviance) on a model-to-model basis (for the model outputs, see Table 4). The degrees of freedom of these chi-square tests is the difference in the number of model parameters between the two tested models. We added effects one at a time, and if the model fit improved at a statistically significant level, then we deemed that effect significant. Notably, the model terms in this analysis are in log-odds units rather than the probability scale (i.e., probability of passing the hands-on test). Where appropriate, we convert these log-odds outcomes to probability scale to aid interpretability of the results.
Note. Fixed and random effect values are model coefficients (β) in log-odds units. Statistics on model fit (residual deviance) reflect the change in deviance from one model to the next, with lower values indicating better fit.
We started with a null model, which included only an intercept and no fixed or random effects. We then added a random effect of instructor team, which significantly improved model fit, X2(1) = 99.08, p < .001, confirming significant variation in performance across teams. Next, we added TDY status as a fixed-effects predictor, which was also significant, X2(1) = 40.43, p < .001. Soldiers who were on TDY (M = 92%) passed their tests at a higher rate than those who were not (M = 85%). There was an effect of soldier rank, X2(1) = 59.80, p < .001, and a TDY-by-rank interaction, X2(1) = 3.92, p = .048. For enlisted soldiers, those who were on TDY (M = 89%) significantly outperformed those who were not (M = 77%), but the same was not true for officers (M = 92% and 93%, respectively). There was an effect of group, X2(1) = 8.58, p = .003, but none of the two-way or three-way interactions with group were significant (ps > .36). To quantify the effect of group, we calculated estimated marginal means that were weighted according to characteristics of the entire sample (e.g., both group means were weighted assuming 14% of soldiers were both enlisted and on TDY, which was the overall sample average across groups). As shown in Table 5, the advantage of the treatment group (M = 87.41%) over the control group (M = 81.75%) was 5.66 percentage points, which was 2.03 points smaller than the raw data means that did not account for differences between groups in the variables of interest.
Note. The raw pass rates do not account for any of the intergroup confounding variables (i.e., differences in instructor team representation, average soldier student rank, and average soldier student TDY status). The model-adjusted pass rates are the estimated marginal means of the final mixed-effects model (on the probability scale), which takes all three variables into account.
Discussion
The results of this experiment suggest that the practical exercises should be made more like actual testing conditions by (1) using only loads rigged with deficiencies and (2) incorporating time pressure. After accounting for differences in sample composition between the control and treatment groups (e.g., rank composition), the two changes to the practical exercises resulted in a 5.66% increase in sling load pass rates. This increase was achieved at essentially no additional investment of time or resources. This seemingly modest increase in pass rate scales up to a significant impact across an entire year of air assault courses. We observed an average class size of 153 soldiers, and we would expect approximately 125 of those soldiers (81.75%) to pass the sling load inspection test with the traditional practical exercises. With the modified practical exercises, we would expect approximately 134 soldiers (87.41%) to pass that portion of the class, an increase of nine soldiers. The Sabalauski Air Assault School conducts about 40 air assault classes per year, meaning that the modified practical exercises would lead to roughly 360 more soldiers passing their sling load inspection annually. The modified practical exercises would therefore result in an increase of about 2.88 classes worth of sling-load test graduates (i.e., 360/125). Increasing pass rates at the air assault course represents a force multiplier, both directly through increasing the number of air assault certified soldiers and indirectly by opening up space for more soldiers to take the course. Critically, the increases in pass rates that we observed in the present study were accomplished without modifying the long-established Army standards.
Limitations
One limitation of the present experiment was that although all six of the treatment classes only used dirty loads during the practical exercises, only four of those classes incorporated the element time pressure. It is not possible to determine the separate and joint contributions of each change. Nevertheless, we do suspect that replacing the clean loads with dirty loads made the larger contribution to the increased pass rate. After implementing the change in load type, pass rates increased and remained stable with the addition of time pressure. Of course, future work would be needed to resolve these questions. Another limitation is that we could not examine performance on the individual loads with an adequate level of precision due to gaps in the data set. It is conceivable, for example, that the changes to the practical exercises affected some loads more than others (e.g., preferentially improved the easiest or hardest).
Future Directions
The learning sciences can be applied to other areas of the air assault course. Practice testing, spacing, and interleaving can be incorporated into classroom activities and/or review materials for use outside of the classroom. We investigated the latter option in another research study, which involved deploying learning content through a web-based and mobile learning platform (Craig et al., 2023). Within the classroom, the lectures could be periodically punctuated by small practice tests or brief review of previously introduced content (i.e., spacing). Of course, these types of interventions could be applied to any other course that requires fact-based learning and/or physical skills. For these categories of learning interventions, there are many potential ways to implement them, which can have measurable impacts on outcomes (e.g., the type and/or timing of feedback during practice testing; e.g., Maddox et al., 2003; Pashler et al., 2007). Moreover, these techniques can be combined with other types of learning techniques, like elaborative encoding (e.g., creating links with old knowledge or generating memory mnemonics; Levin, 1988; McDaniel, 2023) or fading (sequencing material by level of difficulty; Pashler & Mozer, 2013).
Low-lift learning science interventions can also be applied to other Army schoolhouse settings. Of course, the results of our present work are most directly relevant to similar tasks trained elsewhere, like equipment inspection at the Advanced Airborne School (i.e., jumpmaster personnel inspection). That said, given that these techniques have been successful across a wide range of disparate tasks in civilian populations (e.g., radiology, art history, basketball), we have little reason to doubt the same would be true for cases of military application. For example, air defense artillery airframe identification involves categorizing different types of aircraft based on the noises they produce. As with the visual domain, learning auditory discrimination benefits from interleaved learning sequences due to similar cognitive mechanisms (see Chen et al., 2015; Wong et al., 2020; Wong et al., 2021). The results of the present experiment would therefore likely extend to that context and possibly much less similar tasks.
One potential challenge of integrating effective learning science techniques into Army education settings is a common metacognitive illusion. Namely, the use of effective learning techniques often causes people to feel less confident in their learning outcomes than less effective alternatives (e.g., Roediger & Karpicke, 2006). This is likely because the more effective techniques tend to be harder, forcing learners to become aware of gaps in their knowledge that less demanding techniques, like rereading notes, would not. Consequently, learners sometimes prefer the less-effective alternative because they falsely construe it as superior (Karpicke, 2009). For this reason, Army educators should consider educating soldiers about this metacognitive conundrum and inform them that difficulties experienced during learning process are often signs of progress, not evidence of failure.
Working with the schoolhouses, as opposed to the course proponent, has advantages and disadvantages. The main advantage is that implementing these relatively minor changes to Army courses only requires the commander’s discretion as they are not changes in the program of instruction. In addition, working with the schoolhouse leadership and cadre directly affords an opportunity to increase buy-in, which in turn can increase the probability of a successful outcome. However, there are two major disadvantages that should be considered: (1) future schoolhouse leadership can just as easily undo any course modifications, and (2) any potential changes to a course must not conflict with the program of instruction (i.e., the curriculum that is designed by the proponent). For these reasons, the proponent would be an important stakeholder for similar future research efforts.
Incorporating the findings of the present study into the training and education of future instructors and curriculum developers will aid the dissemination throughout the enterprise, regardless of location or proponent. The Common Faculty Development Instructor Course and the Common Faculty Development Developer Course, both taught by the Army University, could be additional areas to translate research findings to improve the quality of output in instruction, lesson plans, and curriculum design that directly impacts student outcomes across the Army Learning Enterprise.
Acknowledgments
Research was sponsored by the U.S. Army Combat Capabilities Development Command and was accomplished under Cooperative Agreement Number W911QY-19-2-0003. The opinions expressed herein are those of the authors and do not reflect those of the U.S. Army. The U.S. government is authorized to reproduce and distribute reprints for government purposes notwithstanding any copyright notation hereon.
References
Adesope, O. O., Trevisan, D. A., & Sundararajan, N. (2017). Rethinking the use of tests: A meta-analysis of practice testing. Review of Educational Research, 87(3), 659–701. https://doi.org/10.3102/0034654316689306
Bates, D., Mächler, M., Bolker, B., & Walker, S. (2014). Fitting linear mixed-effects models using lme4. Journal of Statistical Software, 67(1), 1–48. https://doi.org/10.18637/jss.v067.i01
Bjork, R. A. (1994). Memory and metamemory considerations in the training of human beings. In J. Metcalfe & A. Shimamura (Eds.), Metacognition: Knowing about knowing (pp. 185–205). MIT Press. https://doi.org/10.7551/mitpress/4561.001.0001
Bjork, R. A., & Bjork, E. L. (2020). Desirable difficulties in theory and practice. Journal of Applied Research in Memory and Cognition, 9(4), 475–479. https://doi.org/10.1016/j.jarmac.2020.09.003
Blaxton, T. A. (1989). Investigating dissociations among memory measures: Support for a transfer-appropriate processing framework. Journal of Experimental Psychology: Learning, Memory, and Cognition, 15(4), 657–668. http://doi.org/10.1037/0278-7393.15.4.657
Cepeda, N. J., Pashler, H., Vul, E., Wixted, J. T., & Rohrer, D. (2006). Distributed practice in verbal recall tasks: A review and quantitative synthesis. Psychological Bulletin, 132(3), 354–380. https://doi.org/10.1037/0033-2909.132.3.354
Chen, R., Grierson, L., & Norman, G. (2015). Manipulation of cognitive load variables and impact on auscultation test performance. Advances in Health Sciences Education, 20(4), 935–952. https://doi.org/10.1007/s10459-014-9573-x
Craig, S. D., Riddle, D. L., Lauer, S., Hughes, G. I., Elmore, W. R., Udell, C. E., Murphy, J. S., & Milham, L. M. (2023, April). Investigating the impact of mobile microlearning and self-regulated learning support on soldiers’ self-efficacy and retention within an Army schoolhouse. Journal of Military Learning, 7(2), 29–45.
Delaney, P. F., Verkoeijen, P. P. J. L., & Spirgel, A. (2010). Spacing and testing effects: A deeply critical, lengthy, and at times discursive review of the literature. In B. H. Ross (Ed.), The psychology of learning and motivation: Advances in research and theory (Vol. 53, pp. 63–147). Elsevier Academic Press.
Ebbinghaus, H. (1885). Über das gedächtnis: Untersuchungen zur experimentellen psychologie [Memory: A contribution to experimental psychology]. Duncker & Humblot.
Firth, J., Rivers, I., & Boyle, J. (2021). A systematic review of interleaving as a concept learning strategy. Review of Education, 9(2), 642–684. https://doi.org/10.1002/rev3.3266
Gagné, R. M. (1950). The effect of sequence of presentation of similar items on the learning of paired associates. Journal of Experimental Psychology, 40(1), 61–73. https://doi.org/10.1037/h0060804
Goldstone, R. L. (1996). Isolated and interrelated concepts. Memory & Cognition, 24(5), 608–628. https://doi.org/10.3758/BF03201087
Goode, S., & Magill, R. A. (1986). Contextual interference effects in learning three badminton serves. Research Quarterly for Exercise and Sport, 57(4), 308–314. https://doi.org/10.1080/02701367.1986.10608091
Guzman-Munoz, F. J. (2017). The advantage of mixing examples in inductive learning: A comparison of three hypotheses. Educational Psychology, 37(4), 421–437. https://doi.org/10.1080/01443410.2015.1127331
Hall, K. G., Domingues, D. A., & Cavazos, R. (1994). Contextual interference effects with skilled baseball players. Perceptual and Motor Skills, 78(3), 835–841. https://doi.org/10.1177/003151259407800331
Hughes, G. I., & Thomas, A. K. (2021). Visual category learning: Navigating the intersection of rules and similarity. Psychonomic Bulletin & Review, 28(3), 711–731. https://doi.org/10.3758/s13423-020-01838-0
Kang, S. H. K., & Pashler, H. (2012). Learning painting styles: Spacing is advantageous when it promotes discriminative contrast. Applied Cognitive Psychology, 26(1), 97–103. https://doi.org/10.1002/acp.1801
Karpicke, J. D. (2009). Metacognitive control and strategy selection: Deciding to practice retrieval during learning. Journal of Experimental Psychology: General, 138(4), 469–486. https://doi.org/10.1037/a0017341
Karpicke, J. D., & Blunt, J. R. (2011). Retrieval practice produces more learning than elaborative studying with concept mapping. Science, 331(6018), 772–775. https://doi.org/10.1126/science.1199327
Karpicke, J. D., & Smith, M. A. (2012). Separate mnemonic effects of retrieval practice and elaborative encoding. Journal of Memory and Language, 67(1), 17–29. https://doi.org/10.1016/j.jml.2012.02.004
Kornell, N., & Bjork, R. A. (2008). Learning concepts and categories: Is spacing the “enemy of induction”? Psychological Science, 19(6), 585–592. https://doi.org/10.1111/j.1467-9280.2008.02127.x
Kurtz, K. H., & Hovland, C. I. (1956). Concept learning with differing sequences of instances. Journal of Experimental Psychology, 51(4), 239–243. https://doi.org/10.1037/h0040295
Lenth, R. (2020). Emmeans: Estimated marginal means, aka least-squares means. CRAN. https://cran.r-project.org/package=emmeans
Levin, J. R. (1988). Elaboration-based learning strategies: Powerful theory = powerful application. Contemporary Educational Psychology, 13(3), 191–205. https://doi.org/10.1016/0361-476X(88)90020-3
Maddox, W. T., Ashby, F. G., & Bohil, C. J. (2003). Delayed feedback effects on rule-based and information-integration category learning. Journal of Experimental Psychology: Learning, Memory, and Cognition, 29(4), 650–662. https://doi.org/10.1037/0278-7393.29.4.650
McDaniel, M. A. (2023). Combining retrieval practice with elaborative encoding: Complementary or redundant? Educational Psychology Review, 35(3), Article 75. https://doi.org/10.1007/s10648-023-09784-8
Morris, C. D., Bransford, J. D., & Franks, J. J. (1977). Levels of processing versus transfer appropriate processing. Journal of Verbal Learning and Verbal Behavior, 16(5), 519–533. https://doi.org/10.1016/S0022-5371(77)80016-9
Pashler, H., & Mozer, M. C. (2013). When does fading enhance perceptual category learning? Journal of Experimental Psychology: Learning, Memory, and Cognition, 39(4), 1162–1173. https://doi.org/10.1037/a0031679
Pashler, H., Rohrer, D., Cepeda, N. J., & Carpenter, S. K. (2007). Enhancing learning and retarding forgetting: Choices and consequences. Psychonomic Bulletin & Review, 14(2), 187–193. https://doi.org/10.3758/BF03194050
R Core Team. (2022). R: A language and environment for statistical computing. R Foundation for Statistical Computing. https://www.R-project.org/
Roediger, H. L., III, & Karpicke, J. D. (2006). Test-enhanced learning: Taking memory tests improves long-term retention. Psychological Science, 17(3), 249–255. https://doi.org/10.1111/j.1467-9280.2006.01693.x
Wong, S. S. H., Chen, S., & Lim, S. W. H. (2021). Learning melodic musical intervals: To block or to interleave? Psychology of Music, 49(4), 1027–1046. https://doi.org/10.1177/0305735620922595
Wong, S. S. H., Low, A. C. M., Kang, S. H. K., & Lim, S. W. H. (2020). Learning music composers’ styles: To block or to interleave? Journal of Research in Music Education, 68(2), 156–174. https://doi.org/10.1177/0022429420908312
Footnotes
1 We treated soldier rank as a binary variable (enlisted = 0, officer = 1) to avoid an excessive number of model terms and convergence issues.
Gregory Hughes is a research psychologist at the U.S. Army Combat Capabilities Development Command Soldier Center at Natick, Massachusetts. Hughes obtained a PhD in experimental psychology from Tufts University and has been conducting Army research for eight years. His main research efforts focus on optimizing the acquisition and retention of new knowledge and complex skills.
Shanda Lauer is a research psychologist in the Institutional Research and Assessment Division, Vice Provost of Academic Affairs, at the Army University in Fort Leavenworth, Kansas. She holds a master’s degree focused in discipline-based education research and a PhD in psychology with a neuroscience emphasis. Her program of research focuses on improving communication in the Army and enhancing education through technology use and the application of best practices.
Wade R. Elmore is a research psychologist at the U.S. Army Combat Capabilities Development Command Soldier Center at Natick, Massachusetts. In his 10 years working for the U.S. Army, has worked at the Center for Army Leadership, The Army University, and in 2021 joined the Cognitive Sciences and Applications Team of Combat Capability Development Command Soldier Center. He has contributed to the enterprise level understanding of Army leadership and Army professional military education using Army-wide surveys. Currently, he is engaged in research examining the use of learning sciences best practices on military education and training, and the efficacy of applying these best practices in classroom instruction and through distributed asynchronous training and education platform, characterizing soldier-relevant cognitive and physical traits, and characterizing tactical performance during sustained live-fire exercises.
Back to Top