Leveraging Multi-Domain Military Deception to Expose the Enemy in 2035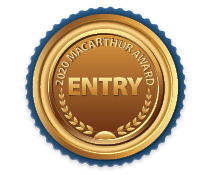
Lt. Col. Stephan Pikner, PhD, U.S. Army
Download the PDF 
The operational problem facing the Army in the year 2035 will fundamentally differ from problems it has previously confronted. The legacy challenge for which the Army’s current platforms and doctrine are still optimized was a problem solved by breaking the Soviets’ second echelon of assault forces with precision long-range fires, fixed-wing air interdiction, and deep strikes by rotary-wing attack aviation. Today, and more so in 2035, the United States’ emerging great-power competitors pose an entirely different challenge. By threatening U.S. access into a theater and denying the assembly areas needed to stage for a decisive counterattack, U.S. adversaries have undercut America’s preferred, expeditionary way of war. This anti-access/area denial (A2/AD) approach hinders the ability to effectively respond to rapid, limited aggression, which leaves allies and partners vulnerable to a wide range of coercive and subversive activities.1 Central to A2/AD is a well-defended, redundant, and largely hidden network of sensors and shooters that can locate, target, and strike friendly forces moving into and staging within a theater of operations.2 To meet this challenge, the Army must adopt a novel approach to finding and fixing the critical components of an adversary’s A2/AD complex to ensure freedom of action in 2035.
Finding the key nodes of an adversary’s A2/AD network in 2035 requires an inversion of the traditional logic of reconnaissance. While cavalry squadrons and regiments can effectively fight for information on the disposition of advancing enemy echelons, finding the critical components of an integrated A2/AD complex is an altogether different issue. Rather than exposing vulnerable friendly forces as they methodically seek out a largely static and well-camouflaged adversary with fire and maneuver, future land forces can provoke an opponent into unmasking the long-range sensor and strike assets central to its A2/AD system by leveraging multi-domain military deception. In particular, this stimulation of an adversary’s targeting and strike complex must consider how artificial intelligence (AI)-informed decisions will be made. In the near future, America’s opponents will likely use such automated systems to fuse a wide range of information into targeting proposals for human decision-making. By triggering the premature activation and deployment of an adversary’s high-value assets in its attempt to find, fix, and strike phantom American targets, multi-domain military deception can be central to an integrated effort to find and destroy the enemy on future battlefields.
This argument for multi-domain military deception as central to finding U.S. adversaries on the battlefields of 2035 unfolds in three parts. First is a brief doctrinal background on military deception as it stands today. Second, and more comprehensively, is a discussion of the probable evolution of adversary A2/AD systems, with a focus on the strengths and potential weaknesses of AI support to targeting. Third is a series of recommendations the Army should consider to best employ multi-domain deception to find the enemy in 2035, with great-power oriented field armies as the integrator for these activities.
Doctrinal Background on Military Deception
The doctrinal and historical background for military deception is well established. Broadly speaking, military deception activities “are planned and executed to cause adversaries to take actions or inactions that are favorable to the commander’s objectives.”3 In the specific context of stimulating an adversarial A2/AD system, this involves amplifying signatures of decoy units and continuously substituting the signatures of real units with simulated ones, thereby overloading an adversary with an overwhelming number of false positives.4 This approach of generating a large number of false positives—the impression of targets when in fact there are none—contrasts with the traditional notion of camouflage, which attempts to create a false negative of no target by masking the signatures of friendly forces. Central to the success of deception efforts is their multi-domain character; in an era of increasingly widespread, sophisticated, and varied sensors, spoofing only one type does little against an adversary capable of rapidly fusing multiple sources of information. “Multi-domain deception,” as proposed by Christopher Rein, “requires close and careful coordination across the warfighting domains to ensure that lapses in one do not undo efforts in other areas.”5
The Probable Evolution of Adversary A2/AD Systems
Gaining an accurate understanding of an opponent’s A2/AD architecture involves integrating information gathered through a variety of means. Overreliance on a single method, such as intercepted electronic communications or overhead imagery, can result in unbridgeable gaps in understanding. The United States has long been unmatched in its battlefield awareness, but its great-power competitors are rapidly gaining ground due to a pair of interrelated developments. First, the increased sophistication, fidelity, affordability, and variety of sensors have made gathering militarily relevant information easier and cheaper. Turning that information into understanding, however, requires a second step, and its impending automation may prove to be revolutionary. The promise of machine learning to fuse raw information rapidly and accurately into actionable targeting proposals will greatly complicate the tasks of hiding—and surviving—on the future battlefield.
Widespread advances in low cost, off-the-shelf platforms and sensors such as drones and high-resolution cameras alongside near real-time, open-source information such as social media posts and commercially available satellite imagery have transformed both the scale and fidelity of information available and the number of international actors who have access to it. Previously only available to leading powers, such sensors have proliferated widely in the past decades. This trend shows no sign of abating; as the means of detection become cheaper, more reliable, and capable of gathering high-quality information, the information advantage enjoyed by the United States for the past several decades will erode further.6
Increasing the diversity and quality of information gathering means solves one half of the challenge. The second half—fusing information from multiple sources to paint a comprehensive portrait of a target—is a more challenging task. Currently, this is a labor-intensive process involving cross-functional teams of analysts painstakingly poring over massive quantities of data captured by increasingly high-resolution sensors. By one estimate, it would take “eight million people just to analyze all of the imagery of the globe that will be generated in the next twenty years.”7 Advances in machine learning, however, may significantly improve and accelerate the fusion of gathered information. Machine-learning classifiers, which “take an input sample and identify it as one of several output classes,” are particularly well suited to fusion and targeting.8 In an AI support to A2/AD targeting context, the input sample would be data gathered through a range of sensors, and the output classes would be a classification of the target. A properly trained machine-learning algorithm with access to a wide range of accurate data would be then able to find the proverbial needle in the haystack and accurately classify a target, greatly accelerating and improving the hitherto laborious information fusion process.9
Much like its diminishing edge in sensors, the United States will not have a monopoly on these automated fusion techniques. By 2035, U.S. adversaries will likely have leveraged machine-learning techniques to fuse information gathered from a wide array of sensors to target their A2/AD weapons. This will present a novel set of challenges in how friendly forces conceal themselves. The wholesale collection of a wide range of signatures of friendly forces may nullify friendly efforts to camouflage in a monodimensional way. For example, minimizing electromagnetic emissions may have a negligible effect against an adversary that can still detect a unit’s thermal, civilian contracting, or social media signature. In more general terms, creating a cohesive false negative against a highly sensitive, multi-domain sensor system will be almost impossible—the adversary will detect something, and well-trained AI will be able to extrapolate an accurate picture of the target from what is detected.
While daunting, this potential revolution in U.S. adversary’s information-gathering and fusion techniques presents an opportunity for friendly forces to find the enemy in the battlefields of 2035. If done cohesively, novel multi-domain military deception can warp an adversary’s algorithms and exploit organizational and procedural tensions between machine-learning-produced proposals and human decision-makers. This deception is not an end unto itself; to clarify the uncertain and contradictory targeting decision information, an adversary will be forced to expose its A2/AD architecture by using increasingly active means that emit unambiguous signatures. Deceiving an adversary into exposing critical nodes of its A2/AD architecture is central to finding well-hidden enemy forces in 2035.
Machine learning is not impervious to spoofing. Machine learning relies more heavily on readily quantifiable data as inputs than existing processes in which humans can place ambiguous evidence in context. Sensors narrowly focused on detecting specific, measurable electromagnetic, acoustic, thermal, gravitational, visual, vibrational, geotagged social media, or computer-aided text analysis data must feed cleanly into a machine-learning algorithm. This algorithm, in turn, is trained by forming correlations between similar signatures and known target characteristics.10 Its accuracy hinges on the richness of its training dataset, where true positives and valid, associated covariates form a basis for the algorithm to be tuned and updated. In a military context, the true positives would be actual cases of the target, and the associated covariates would be the full range of measurable signatures across all domains. Currently, the fusion of multi-domain information happens through manpower-intensive cells on military staffs; machine learning offers the opportunity for this same process to happen rapidly, automatically, and through the recognition of patterns of correlations that may elude human cognition. Deliberately muddying the waters through military deception operations that obfuscate how a true target looks can undermine this learning process, tricking an AI-enabled A2/AD system to look in the wrong place for the wrong signatures. Or, as Edward Geist and Marjory Blumenthal put it, friendly forces can employ “fog of war machines” to confuse adversarial sensors and the associated machine-learning processes.11
This increased reliance on quantifiable data streams to feed a machine-learning-driven targeting algorithm can also open a critical vulnerability within an adversary’s organization: it comes at the expense of human expertise and intuition, making the entire system vulnerable to multi-domain deception. The halting, uneven development of AI over the past several decades is littered with examples of seemingly clever machines that, when posed with real-life challenges beyond the narrow scope of their training, are completely baffled.12 In contrast to conventionally programmed systems, there is no team of engineers who can easily tweak the code to better support the human decision-makers in the system but rather a black box where outputs are generated by hidden layers of weighted links within a neural network formed by iterating through training data.13 This lack of clarity as to how the machine learns may cause friction in an AI-enhanced human decision-making system. Prior to a real-world failure, a machine-learning algorithm’s assumed omniscience may diminish the relative value of human decision-making, creating the dilemma that when the machine-learning system is most needed it is least trusted, while the human-driven alternative to it has atrophied in status and capability.14
Deceiving an adversary’s machine-learning-driven targeting system can trick the adversary into either activating high-signature sensors or striking at phantom targets. In future land conflict, this opens an important window of opportunity to deliver friendly joint counterbattery fires against the enemy’s “kill chain” of sensors, command and control nodes, and weapons platforms.15 What multi-domain military deception brings to future warfare is the potential to spoof the machine—to confuse an AI-augmented adversary’s targeting chain—and through that deception, expose its reconnaissance and strike assets.
Recommendations
Developing and fielding the organizations, doctrine, training, and equipment needed for effective employment of multi-domain military deception requires a deliberate and coordinated approach.16 This section outlines four specific considerations for a force capable of leveraging multi-domain deception to find the enemy in 2035. First, the components of an integrated, multi-domain deception posture must be flexible and adaptable to maintain a sustained deception effect against a learning adversary. Second, multi-domain full-spectrum deception cannot begin in a crisis but rather must be grounded in baseline conditions set during competition below the threshold of armed conflict. Third, as it is highly likely that land operations will involve allies and partners fighting alongside U.S. ground forces, multi-domain deception will be enhanced by including them into a theater-wide scheme. Lastly, multi-domain deception must not be viewed as an end unto itself but rather a means to prompt an adversary to “show its hand.” By provoking an enemy’s A2/AD kill chain to pursue phantom formations, multi-domain deception can stimulate—and therefore expose—critical components of its network to destruction.
The first consideration in developing multi-domain deception is the interactive, competitive, and evolutionary dynamic of military deception. Successful deception depends as much on an adversary’s perceptions and interpretations of friendly signatures as it does on the emissions that formations generate. In addition to the technical dimensions of generating credible apparitions, there is a critical organizational element that is grounded in the U.S. adversary’s military culture: what may fool Americans may not spoof an adversary, and methods that may be effective against one competitor may be discounted by another. Deception efforts must continuously adapt as adversary biases, capabilities, and doctrine evolve.
Second, successful deception in a crisis of conflict must be built on a foundation established in peacetime. Persistent competition below the threshold of armed conflict should include deliberate efforts to monitor, mask, and simulate the full spectrum of friendly land force signatures. The goal of this is twofold: first, to comprehensively “see ourselves” and second, to influence the training data sets that U.S. adversaries are building on friendly forces in peacetime to train their AI targeting systems. To achieve these goals, friendly formations operations in peacetime must be thoroughly monitored by teams tasked with building a comprehensive profile of a unit’s signatures and emissions. This profile will be the baseline of what can be detected and exploited by an adversary’s A2/AD sensors. These teams would monitor friendly forces in both simulated tactical engagements and during deployment to real-life forward locations. From this data, gathered in peacetime competition during rotational deployments and exercises, a thorough, all-spectrum picture of how land formations appear to the full range of an adversary’s sensors can be painted.
That comprehensive signature of friendly forces catalogued in peacetime can be used in two distinct ways. The first is to mask the footprint of true formations by minimizing their emissions. Contrary to the conventional wisdom of “train as you fight,” many of the steps that would be taken to mask a unit’s footprint should only be taken in a real-world crisis. Exercising them routinely during peacetime competition would allow an adversary to learn alternate “tells” of a unit’s location and disposition that are harder (or impossible) to mask during conflict. For example, minimizing a unit’s electromagnetic footprint during a rotational deployment may drive an adversary to search more closely for other, less easily concealable signatures as key indicators of friendly forces.
In addition to informing how best to mask the true location of a friendly unit in crisis, the comprehensive signature of friendly forces can be replicated as a deception technique. This signature not only includes the military equipment of a friendly formation but also the social media and commercial contracting emissions that are produced by the deployment of such a force. Friendly deception units that can simulate the characteristics of full combat formations can act as “honey pots” that draw attention away from actual formations and fool the enemy into exposing critical components of its A2/AD kill chain.
Third, future warfare in the land domain is almost guaranteed to take place in a coalition context. To maximize the tactical effectiveness of multi-domain military deception, the signatures of allied and partner land formations should be measured and mimicked in a manner similar to American ground forces. At the theater level, this includes military deception operations involving ports of debarkation, strategic force hubs, and other critical infrastructure that enables friendly forces to surge into an area of operations. As these facilities are often near population centers and typically have dual civilian and military functions, special consideration must be given to allied concerns about and constraints on military deception activities. Clear lines reinforcing the protected status of certain facilities and personnel (e.g., hospitals, religious sites, medical personnel) must be drawn and communicated with U.S. allies to avoid any perception that these efforts would violate the Law of Armed Conflict.17
Finally, the overarching purpose of this multi-domain military deception effort is to find the enemy on the battlefields of the future. It is in presenting an irresistible, but false, target to the adversary where multi-domain military deception facilitates finding the enemy. Stimulating the enemy’s integrated system of sensors and shooters by simulating the presence of lucrative, but phantom, targets can expose the high value, highly survivable assets in their kill chain. Effective deception can trigger a full range of adversary sensors—reconnaissance teams, electronic attack systems, satellites, unmanned aerial vehicles, ground surveillance radars, and cyber assets to activate in search of a chimera. An enemy’s A2/AD weapons such as theater ballistic missiles, long-range artillery, and special forces would similarly deploy from secure, camouflaged sites to strike what they believe are actual friendly concentrations. Anticipating this activation, friendly intelligence, surveillance, and reconnaissance systems, synchronized with the multi-domain military deception plan, can anticipate, sense, and exploit this overt and active enemy activity. Instead of an ineffective and costly search against hardened and camouflaged components of an A2/AD system, multi-domain military deception can trick our future adversaries into exposing themselves prematurely.
Implementing these recommendations requires detailed understanding of a great-power competitor, the proper level of friendly authorities and capabilities, and the posture during competition below the threshold of armed conflict to maintain and modulate an enduring deception campaign. In the Army’s current structure, this task would most likely fall between the corps and the Army Service component command. As the Army adapts to great-power competition, the final recommendation of this article is that a field army, focused on competing against a specific adversary, should be the proponent for and integrator of multi-domain military deception operations.18 Unburdened of the theater-wide responsibilities of the Army Service component command, and in contrast to a corps oriented on a specific adversary in peacetime competition, a field army would be best positioned to design and prosecute an enduring, cohesive, and tailored military deception campaign. Through this deception, the Army can force its adversaries to strike out blindly against shadows, exposing the critical components of their A2/AD architecture to detection, destruction, and ultimately, defeat.
Notes
- Andrew J. Duncan, “New ‘Hybrid War’ or Old ‘Dirty Tricks’? The Gerasimov Debate and Russia’s Response to the Contemporary Operating Environment,” Canadian Military Journal 17, no. 3 (Summer 2017): 6–11.
- Wilson C. Blythe Jr. et al., Unclassified Summary of the U.S. Army Training and Doctrine Command Russian New Generation Warfare Study (Fort Leavenworth, KS: Army University Press, 2020), accessed 20 October 2020, https://www.armyupress.army.mil/Portals/7/online-publications/documents/RNGW-Unclassified-Summary-Report.pdf?ver=2020-03-25-122734-383.
- Field Manual 3-13.4, Army Support to Military Deception (Washington, DC: U.S. Government Publishing Office, 2019), 1-2.
- Ibid., 1-8.
- Christopher M. Rein, ed., “Multi-Domain Deception,” in Weaving the Tangled Web: Military Deception in Large-Scale Combat Operations (Fort Leavenworth, KS: Army University Press: 2018), 2.
- Michael C. Horowitz, The Diffusion of Military Power: Causes and Consequences for International Politics (Princeton, NJ: Princeton University Press, 2010).
- Christian Brose, The Kill Chain: Defending America in the Future of High-Tech Warfare (New York: Hatchette, 2020), 59.
- Patrick McDaniel, Nicolas Papernot, and Z. Berkay Celik, “Machine Learning in Adversarial Settings,” IEEE Security & Privacy 14, no. 3 (May 2016): 68–72.
- Stephan Pikner, “Training the Machines: Incorporating AI into Land Combat Systems,” Landpower Essay Series (Washington, DC: Institute of Land Warfare, January 2019), accessed 20 October 2020, https://www.ausa.org/sites/default/files/publications/LPE-19-1-Training-the-Machines-Incorporating-AI-into-Land-Combat-Systems.pdf.
- Gary Marcus, “Deep Learning, a Critical Appraisal” (paper, New York University, 2018), accessed 20 October 2020, https://arxiv.org/ftp/arxiv/papers/1801/1801.00631.pdf.
- Edward Geist and Marjory Blumenthal, “Military Deception: AI’s Killer App?,” War on the Rocks, 23 October 2019, accessed 20 October 2020, https://warontherocks.com/2019/10/military-deception-ais-killer-app/.
- Marcus, “Deep Learning, a Critical Appraisal.”
- McDaniel, Papernot, and Celik, “Machine Learning in Adversarial Settings.”
- Peter Hickman, “The Future of Warfare Will Continue to Be Human,” War on the Rocks, 12 May 2020, accessed 20 October 2020, https://warontherocks.com/2020/05/the-future-of-warfare-will-continue-to-be-human/.
- Brose, The Kill Chain.
- Eric Wesley and Jon Bates, “To Change an Army—Winning Tomorrow,” Military Review 100, no. 3 (May-June 2020): 6–18.
- “Geneva Convention (IV): Relative to the Protection of Civilian Persons, Part I,” Infoplease, 12 August 1949, accessed 2 November 2020, https://www.infoplease.com/primary-sources/government/united-nations/convention-relative-protection-civilian-persons-time-war.
- Amos C. Fox, “Getting Multi-Domain Operations Right: Two Critical Flaws in the U.S. Army’s Multi-Domain Operations Concept,” Land Warfare Paper 133 (Washington, DC: Association of the United States Army, June 2020), accessed 20 October 2020, https://www.ausa.org/sites/default/files/publications/LWP-133-Getting-Multi-Domain-Operations-Right-Two-Critical-Flaws-in-the-US-Armys-Multi-Domain-Operations-Concept.pdf.
Lt. Col. Stephan Pikner, PhD, U.S. Army, is an Army strategist (FA59) and a graduate of the Advanced Strategic Policy and Planning Program. He holds a BS from the U.S. Military Academy, an MPA from the Harvard Kennedy School of Government, and a PhD from Georgetown University. His most recent assignment was as the deputy G5 (plans) of NATO Allied Land Command in Izmir, Turkey.
Back to Top