Leveraging Data for Warehouse Distribution Success During Operation Allies Welcome
A Retrospective on Operationalizing Data
Maj. Daniel Marcey, U.S. Army
Download the PDF 
The soldiers of the 2nd Armored Brigade Combat Team, 1st Armored Division (2/1AD) received the first 322 of 11,427 Afghan evacuees at the Doña Ana Village, New Mexico, on 21 August 2021. Led and supported by the entirety of “Team Bliss,” 2/1AD assisted the Afghan resettlement effort for 132 days.1 In all, elements of the division, intergovernmental organizations, and many nongovernmental organizations worked together to resettle asylum seekers in the United States.2 The operation was remarkable in purpose and effort, and the division’s planning phases, execution, and lessons learned are available in a multitude of professional publications.3 Many of these assessments cover the breadth of the operation. To offer a substantively different perspective, this review focuses on the framework and methodology employed by a company headquarters to leverage data to support the distribution of the provisions, donations, and government-purchased aid required to meet and sustain the needs of those being resettled. Drawing from theoretical and practical sources, this review alternates between technical writing and storytelling to explain complex topics in an engaging and informative narrative. Upon reading, practitioners will have a reference point to understand and apply emerging data-intensive solutions inherent to twenty-first-century operations.
Bottom Line Up Front
By comparing the principles of data strategy and examining the framework and methodology employed by a company headquarters to leverage data, this review serves as a foundation for future leaders to create, acquire, and manage data. Additional lessons include the fact that data tools do not have to be technologically sophisticated, they do not have to be expensive science projects, and data tools created by those at the threshold of execution can manage adaptive solutions. Commands must prioritize data employment, as in-depth analysis empowers leaders, culminating in shared understanding. Key to championing data is underwriting the residual risk of organizational change during execution. Notably, the risk is prudent, and when data is integrated into the operations process, commanders are better equipped to visualize, describe, and lead their organizations. Armed with these insights, leaders can make faster and better-informed decisions by leveraging data to assess risk, optimize combat power, and fully employ their organization to win.
Receipt of the Mission
On the afternoon of Friday, 1 October 2021, Headquarters and Headquarters Company, 1st Battalion, 35th Armored Regiment, received the warning order to support the Doña Ana Village (DAV) (the operational name for Fort Bliss’s newly formed life support area) distribution warehouse beginning 3 October 2021. The distribution warehouse aimed to coordinate, receive, and provide aid to the Afghan evacuees housed at the DAV. However, by the beginning of October, the stock levels at the distribution warehouse did not reflect the need. Team Bliss had been receiving evacuees for forty days and distributed approximately nine thousand prepackaged aid bags, resulting in shortages of the aid items comprising a complete aid bag. Therefore, considering the total planning population for DAV was ten thousand evacuees, the mission, determinable by reduction, was to estimate the required aid necessary to construct prepackaged aid bags for one thousand Afghans. More specifically, Headquarters and Headquarters Company needed to estimate for, assemble, and provide one thousand prepacked aid bags, referred to by the nongovernmental organizations as “kits,” to a sister battalion whose mission was to distribute the kits during reception and staging.
A Primer on Data Centricity
The future digital Army requires commanders to operationalize data and data analytics. To realize the future, leaders must be held accountable for data management, governance, and analytics. In combination, these data initiatives result in data centricity, which, as an organizational disposition, enables rapid decision-making.4
Secretary of the Army Christine Wormuth described data centricity as “empowering leaders and Soldiers with the right information at the right time to gauge risk, optimize combat power, fully employ national means, and attain decision dominance at all echelons.”5 A data-centric vision is realized as information seamlessly flows through channels accessible in real-time rather than limited to scheduled and specialized data collection points. A data-centric force can more quickly access and understand new data’s relevance, enabling iterative decision-making.6 To hasten the transformation, commanders at all levels must see themselves responsible for realizing the Army’s data goals.
Central to delivering a data-centric organization is a commander’s data strategy. Data strategies are emergent or deliberate. So, no matter the approach, the organization will have a data strategy.7 Commanders who value data will foster a data-centric culture. Conversely, commanders who do not value data will find their reliance on industrial-aged data collection methodologies lacking, and the organization’s data resources will be underutilized. These shortcomings generate increasing organizational technical debt, detracting from winning on the twenty-first-century battlefield.
Framework for Developing a Data Strategy
As done in the reverse planning process, commanders must visualize the effects and outcomes to service the objective and then work backward by implementing policy, dedicating resources, and task-organizing formations to achieve dominance. Describing the framework in technical terms, a data strategy details use cases supported by data products and data assets whose workflows and usage modalities meet the needs of distinct business processes. Therefore, generating use cases is the start of a data strategy. From the use cases, technical specifications define the capabilities of the database. Then, metrics are identified to satisfy the capabilities. Commanders must also implement data governance to manage the inputs and outputs and ensure activities are supported by adequate resourcing.8
Data Products
Next, commanders are tasked with developing solutions for the use cases, called data products, which require constant organizational effort to create and maintain. Even the best-designed products, requiring low or no user input, consume organizational resources, and their elicitation requires a well-planned data product strategy. A data product strategy is an optimized value chain generated from patterned activities to create a data artifact (see the figure). Further demystifying the technical jargon requires an explanation of a data artifact. A data artifact is a digital product derived from a subset of a data source. It is created by an intelligent agent, either a human or software, by performing a unique function on the data source. In simpler terms, a data set sources, defines, baselines, and leverages data for decision-making.9 In a sophisticated strategy, the data source is continually mined, and the baseline is updated. This repetition is the beginning of machine learning models.
Data Assets
Organizations create data assets from well-formulated data strategies and optimally designed data products. Data assets are highly sought-after models, dashboards, reports, and interfaces enabling decision-making.10 Not discounting the effort required to create a data asset, the most significant organizational resource consumption occurs here at the data-human interface. On average, our ability to understand or operationalize the data is less than our ability to gather or aggregate the data.11 The inefficiency is primarily attributable to suboptimal user experience. Alternatively, an experienced user can rapidly consume the “nth” order trends, discern higher-order observations, and infer value beyond the display—achieving predictive, prescriptive, or semantic levels of understanding.12 Put plainly, users struggle to gain understanding because they lack the experience to visualize the data’s tangible manifestation. Notably, such inexperience is magnified by the users’ well-intentioned but inefficient data product strategy. Much time and effort are lost from the compounding effects of inexperience.
However, occasions to gain experience are abundantly available, and deliberate data strategies mandate data inclusion to capitalize on those occasions. There are many opportunities to lead product delivery teams focused on the continuous improvement, ingestion, and deployment of data sets.13 Moreover, leaders must interact with the underlying data. Following the business process from data ingestion to dissemination, commanders must execute simulated transactions to develop an understanding of programmatic assumptions, case behaviors, and boundary conditions.14 Failing to grasp the limitations can risk results in a misaligned decision. Considering commanders are responsible for organizational alignment, misaligned business intelligence tools are also their responsibility and pose a considerable risk to the mission. Investing in our understanding of the digital systems aiding in decision-making is more critical than understanding the inner workings of our data-adjacent hardware. To correct poorly aligned data and generate value from data centricity, commanders must ensure their organizational data is visible, accessible, understandable, linked, trustworthy, interoperable, and secure (VAULTIS). These seven goals, called VAULTIS, support the Department of Defense’s eight guiding principles:
- data as a strategic asset,
- collective data stewardship,
- data ethics,
- data collection,
- enterprise-wide data access and availability,
- data for artificial intelligence training,
- data for purpose, and
- data designed for compliance.15
Admittedly, this collection of principles and goals gives pause. Determining the essential tasks among those listed requires intimate knowledge of intent throughout the vertical chain of command.
Here again, a data strategy at echelon pays dividends. One of the primary purposes of a command is to discern higher headquarters’ intent for subordinates. Therefore, explaining how and why the complex strategic document applies will remove barriers. In lay terms, data strategies identify how to use raw data, manage bureaucracy through transparency, and emphasize the skills required to create, acquire, clean, manage, and analyze data.16 Finally, strategies include training to enhance practitioners’ data operationalization.
Implementing Data Solutions
Up to this point, the soldiers and the volunteers of Team Rubicon (the NGO leading donation efforts) had been sorting, conducting spot inventories, and packaging donations on a first in, first out basis, maximizing throughput.17 However, the needs of the life support area were maturing, and the rapid but unfocused donation process needed manageable controls.18
This table presents the initial forecast utilized in the distribution warehouse to estimate the number of kits based on gender and size, 2 October 2021.
The initial mission of developing “kits” rapidly expanded into a diverse set of tasks. The variability in persons and provisions precluded the use of a single kit (see table 1). Instead, the distribution warehouse managed thirty-seven size variations. Notably, a single “kit” included a pair of pants, two shirts, socks, undergarments, and a small assortment of toiletries. Children’s kits also included a small stuffed animal. The company headquarters’ first attempt at a data product was realized with these inputs. The data product was rudimentary, but the generalized size chart became the building block for projecting how many kits by size and gender would be required to serve the remaining one thousand evacuees.
Recognizing the practicality of the “powers of ten” approach, we promptly assigned values to illustrate the size distribution across a theoretical population of ten evacuees. Each grouping of ten represents one of the eight major divisions of the dataset—for example, adult-male, male-child, female-toddler, or newborn. However, having no experience clothing one thousand people, the estimate needed an authoritative reference point. Scholarly results on warehousing and managing at distribution points were used to provide an educated estimate and establish a baseline commensurate with an average American distributor. The estimate was then skewed toward smaller sizes after considering the differences in the height and weight of an American versus an Afghan. From the original ratio-based estimate, an estimate generating raw numbers was formulated. The subsequent raw number estimate is a product of DAV’s census data draped across the corresponding demographic’s size chart ratio. The resultant model became the basis for reporting. Notably, the combination of these two models introduced risk. Most consequential was that the recommended plan assumed the previous nine thousand evacuees would be representative of the next one thousand.
Nevertheless, the risk was underwritten, and the resultant model became the basis for reporting. Notably, sizes were weighted with factors of safety (FOS) and increased the planned requirement to reduce the risk. The deliberate effort to assess and mitigate risk is crucial to emphasize for leaders. The probability of model error was likely, and the consequences were moderate. Therefore, the model was a medium risk to the mission. Maintaining “guest goodwill” was an essential task, and linkages between aid and goodwill are evident.19 For these reasons, failure to provide aid poses a clear risk to the mission. With an understanding of the operational environment, a requirements model, and known risk mitigated and underwritten by commanders, a predictive requirement emerged.
Theory Explained Practically
Relating the effort to the principles of data, the first use case was resolving and forecasting the requisite aid. Considering data usage was not a specified or implied task, the data strategy and subsequent use case emerged in response to the evolving needs and circumstances. In fact, the entire process was impromptu, and leaders had no formal training or schooling in data utilization; instead, numerous repetitions, problem-solving principles, and forward-thinking led to many organic decisions. Such a scenario is common among data novices. Ignorant of the industry terms, leaders fail to seek out the most appropriate tools and instead are left re-creating rudimentary single-use data products. Such extraneous effort delays execution and, more importantly, prohibits iterative improvements and limits opportunities to maintain the data or create “net new features.”20 Notably, such a phenomenon is well known and identified in the Army Intelligence Data Strategy; cultivating data literacy is a primary line of effort to overcome the shortfall.21
Notwithstanding such shortfalls, the first use case came with the principal data product, the ratio-based estimate. From the data product, the size report, exported nightly in a situation report, was the data asset. Finally, the human-data interface operationalized the data, apprising the commander. In this specific case, we can observe the entire data product life cycle. However, the considerations that remain for review are necessary to build the data product.
From the outset, the database’s format prioritized data entry. The formatting was prepared in accordance with the inventory procedures to streamline manual data entry. Focusing on acquired data ensured the outputs were limited to the inputs. Specifically, because the tool was initially focused on data collection, the data product was not reliant on any information not primarily available. In a word, the tool measured what mattered, and its success hedged on the core metrics. Yet, with many unknowns, the model was developed to leverage a scalable, flexible formula. Recognizing demographics and the gender-size ratios would drive the data product; the formulary was separated from the aid forecasts and subsequently linked through sheet-to-sheet formularies. The product of these linkages was the primary data artifact. Isolating the data product and the subsequent artifact allowed the holistic model to undergo numerous tests to ensure its behavior was as expected. Such test pages and intermediate outputs confirmed the model’s training and inferencing performed correctly. Taking the time to ensure model behaviors is a must for two reasons. The first is preventing data drift or “nth” order transformations, which result in accuracy breakdowns. Notably, the model, which operated with little sophistication, required intermediate reports as they were the only leading indicators of a data problem. The second is education. The reports taught the creators how the model responded to stimuli and familiarized senior leaders with the model’s theory and risk, generating trust through understanding. Teaching others and generating buy-in was invaluable.
Operationalizing Data Solutions
Armed with an unproven model and an end state in mind, the task at hand was influencing the current scheme of sustainment. In general, Team Bliss had developed a hub-and-spoke distribution model. The distribution warehouse served as the hub. The spokes comprised the various outlets, serving as both enduring points of supply and event-based distribution nodes. For example, the shoe store, infant store, and the “storefront” were enduring locations. Alternatively, pop-up distribution points were used for one-time issuance of items like winter jackets and sleeping bags.
Considering the scheme of sustainment’s level of maturity, the distribution method was well-established. However, as was the case during requirements generation, the initial scheme lacked the deliberate planning common in the later crisis response phases.22 Therefore, instead of spot inventories, which were outdated at the time of recording, the priority was to institute an inventory method accounting for the intake and distribution of aid.
Summarily, it was clear the warehouse needed to change its process immediately, but expecting such rapid change was unwise. In hindsight, any approach should have anticipated resistance to change, necessitating a deliberate strategy to build consensus and gain buy-in. The storming, forming, and norming processes were initially disruptive, producing fewer kits than in previous days. However, by consistently promoting data-driven operations, the organization reached an inflection point, and incremental success fostered widespread buy-in.
Changing the Data Culture
Up to this point, the sustainment scheme’s “problem structure” exhibited characteristics of an “ill-structured adaptive iteration.” In other words, leaders continuously changed requirements to refine the problem’s structure and solution.23 Informal command relationships within the interagency working group magnified the challenges inherent to numerous adaptations. Together, the uncertainties created a considerable amount of organizational churn. Given the issue’s complex, nonlinear, and dynamic nature (providing the right aid at the right time in the correct quantities), the warehousing effort was an ideal opportunity to replace reactive solutions with data-driven forecasting. Overcoming the organizational cultural barrier required consensus, team building, and a deliberate change strategy.
Ultimately, the working group found value in the data, creating many new features. In fact, the number of products overran the organization’s ability to integrate the information, which led to conflicting data. The conflict was the result of nonexistent data governance. In short order, informal agreements between the creators and consumers of the data resolved the issue, and a loosely enforced governance served the organization for the remaining months. Highlighting the challenges associated with disruptive data leadership is not an indictment of an actor or demonstrable grand achievement. Instead, the review presents the complexities of culture change. Commanders change cultures by changing what an organization prioritizes and reinforcing pockets of success. Such was the case within the working group and among the consumers of the data products. From here, data operations focused on maintaining the learning algorithm, generating new features, and normalizing the dataset.
Managing the Data Products Algorithm
The accompanying table illustrates the fluctuations in averages categorized by size and gender. Precisely, table 2 captures the change in consumption rates influenced by reintroducing data reflective of the actual size requirements on DAV. As stated, the model was constructed to accept such information. Crafting the learning model was not without difficulty, especially since the model is hosted in Microsoft Excel. Creating a learning algorithm is often prohibitive in Excel, or at least for the general user. Linking too many interlocking variables together commonly drives Excel into an error. The circular dependency prevents automatic updates. However, the crucial breakthrough in overcoming this limitation was the explicit separation of the constants associated with the data products, particularly the gender-size ratios. Creating an adjacent column, translating the linkages, and manually transcribing the dependent averages allowed the cycle to repeat continuously without falling victim to the prohibited circular dependency.
Once able to forecast requirements, aid was distributed cyclically; the model was trained using results from the largest cycles, improving forecasting capabilities, and this table details the evolution of the model’s controlling ratios.
Reflecting on the averages, numerous observations are readily available. However, in some cases, the cause of the change requires a caveat. The most prominent example is the skew toward larger sizes for adult-female clothing. Through conversation, made more productive via a translator, we discovered the women chose the larger sizes stylistically, preferring more modest forms instead of the more Western concept of fit.
Additionally, eliminating sizes contributed to the ratio redistribution. Choosing to eliminate the sizes was done because either the demand was too low, the supply was too low, or as is the case for 5/6T toddler clothing, it was more common for manufacturers to combine the sizes.
Preventing the tool from driving execution is seemingly obvious, but all too often, we allow the inflexibility of data tools to steer our behavior. This stark reversal in logic is misguided. Moreover, in the case of the most sophisticated tools, digital safeguards are withheld at a higher echelon by the manufacturer or granted to a single super user, which results in users at the lowest level re-creating the valuable functions on their own in separate software. However, since those safeguards were not in place, this tool is an example of the inverse, illustrating the significant impact of allowing users to restructure it fundamentally.
Learning Models Enhance Use Cases
From the original data product and its first two use cases, additional data products and numerous data artifacts were generated, and each data artifact was used to create a data asset. In essence, the model served as the template for similar tools. These additional tools expanded data analysis capabilities far beyond the prepackaged kits. The models were then synthesized and added to the nightly reporting format. In practice, this allowed the model to drive resourcing and economically assign forces to appropriate resupply missions by optimizing daily expenditure rates, forecasting shortages, or identifying opportunities for pop-up distribution points (among other functions). In total, the model facilitated the distribution of more than twenty thousand articles of clothing. While impressive, the aid distribution estimate is much higher since numerous other product types managed at the warehouse were not included in the tally. Left uncounted are diapers, formula, hygiene products, traditional clothing, scarves, shoes, toys, books, jackets, and many other items.
Not a Database
Taking a moment to address data scientists, analysts, or experts, an observation may be accurate. The tool defined by the data it collects, its object-relationships, and its use within Excel does not qualify as a “true database.” Instead, Microsoft Access or Azure would be more suitable as database management systems. However, to this end, such an assertion is not correct. Leaders must conclude that early data adaptations at the tactical level must prioritize inclusion over technological capability, justifiable by recognizing that specialized data collection points are nearly prohibitive and numerous. VAULTIS goals accommodate the substitution.24 Which is to say, based on a unit’s data maturity, data-forward leaders must focus on teaching and improving a unit’s data culture while finding ways to have their work included in current operations. If inclusion requires manual translation, copy-and-pasting screenshots (or the like) for now, so be it. Achieving information dominance takes precedence over strict academic precision.
Analog Data
Numerous articles emphasize the role of data in shaping the common operational picture (COP). These examples often showcase cloud-based tools informed by data insights from business intelligence suites powered by artificial intelligence. These examples represent the extreme cutting edge of data operationalization. Organizations must develop a very mature data culture to implement these solutions, acquire the infrastructure, and specialty train users. Many organizations are not prepared to leverage these tools. Alternatively, commands must find ways to operationalize their data using traditional techniques. To this end, the anecdotes herein are offered as a solution.
Moreover, we must recognize the enduring value of an analog COP. Undoubtedly, the idealized doctrine, organization, training, materiel, leadership and education, personnel, facilities, and policy (DOTMLPF-P) solutions will eventually be integrated into the force, and all soldiers will be sensors and consumers on a networked battlefield. Until then, and perhaps even into the future, we must not underestimate the analog COP. What must change is the interaction between the analog COP and our data systems. Leaders must emphasize a two-fold approach:
- the information on the COP must be, on its own, value-added; and
- the analog COP must effectively inform and be informed by digital data.
The Many Ways Forward
In summary, the transformative role of data in organizational decision-making is realized when leaders embrace data strategies, utilize accessible tools, and integrate data into their operational processes for more effective and informed leadership. In the short term, leaders should prioritize data literacy training, encouraging hands-on use of existing tools. Advanced training programs for sophisticated data analysis should be implemented within the mid-range. In the long term, institutionalize data-driven practices through dedicated roles and continuous learning. Implementing a strategic approach ensures data’s progressive and sustained integration into decision-making processes. Employing organizational resources to leverage data will result in implementing data-intensive solutions crucial for twenty-first-century operations, enabling leaders to assess risk, optimize combat power, and win.
Notes
- Department of Defense Office of the Inspector General (DOD OIG), Management Advisory: DoD Support for the Relocation of Afghan Nationals at Fort Bliss, Report No. DODIG-2022-064 (DOD OIG, 2022).
- Scott Schultz and Anthony Lupo, “From Crisis to Campaign: Operation Allies Welcome at Fort Bliss, Texas,” Military Review Online Exclusive, 9 December 2022, https://www.armyupress.army.mil/Journals/Military-Review/Online-Exclusive/2022-OLE/Schultz/.
- Ibid.; Ian Cobbs, “Finding Love, Acceptance, and Hope While Serving Refugees,” Team Rubicon, 20 June 2024, https://teamrubiconusa.org/news-and-stories/finding-loveacceptance-and-hope-while-serving-refugees/; Alexandra J. Nylen et al., “Then, We Lost Everything”: Afghan Evacuee Experiences of Operation Allies Refuge and Operation Allies Welcome (Center for Human Rights and Humanitarian Studies and the Refugee Dream Center, April 2023), https://watson.brown.edu/chrhs/files/chrhs/imce/research/RDC%20Report_FINAL.pdf.
- Office of the Chief Information Officer (OCIO), Army Data Plan (Department of the Army, 2022), 15, https://api.army.mil/e2/c/downloads/2022/10/13/16061cab/army-data-plan-final.pdf.
- Christine E. Wormuth, quoted in Darren LeBlanc, “Quarterbacking Digital Transformation,” Army AL&T Magazine, Winter 2023, 32, accessed 31 May 2023, https://asc.army.mil/armyalt/Winter2023/html/print/ArmyAL&T_Magazine_Winter2023_DL.pdf.
- Thomas D. Richardson, “Data Centricity and the 1st Cavalry Division’s ‘Speed of Relevance’ during Warfighter 23-04,” Military Review 103, no. 5 (September-October 2023): 107–13, https://www.armyupress.army.mil/Journals/Military-Review/English-Edition-Archives/September-October-2023/Data-Centricity/.
- Tobias Macey and Ranjith Raghunath, hosts, Data Engineering, podcast, episode 397, “Defining a Strategy for Your Data Products,” produced by Boundless Notions, 22 October 2023, https://www.dataengineeringpodcast.com/data-product-strategy-episode-397.
- Ibid.
- Ibid.
- Ibid.
- Ibid.
- Office of Business Transformation, The Enterprise Data Analytics Strategy for Army Business 2018-2022 (Headquarters, Department of the Army, May 2018), https://www.army.mil/e2/downloads/rv7/professional/enterprise_analytics_strategy.pdf.
- Macey and Raghunath, “Defining a Strategy for Your Data Products.”
- Ibid.
- Ibid.
- Erik Davis, “The Need to Train Data-Literate U.S. Army Commanders,” War on the Rocks, 17 October 2023, https://warontherocks.com/2023/10/the-need-to-train-data-literate-u-s-army-commanders/.
- Schultz and Lupo, “From Crisis to Campaign.”
- Ibid.
- Ibid.
- Macey and Raghunath, “Defining a Strategy for Your Data Products.”
- Orlando Nieves III, John Boyer, and Feihrren Calhoun, “Take Ownership of Your Formation’s Data Literacy,” Military Review Online Exclusive, 25 August 2023, https://www.armyupress.army.mil/journals/military-review/online-exclusive/2023-ole/data-literacy/.
- Schultz and Lupo, “From Crisis to Campaign.”
- Army Techniques Publication 5-0.1, Army Design Methodology (U.S. Government Publishing Office, 2015), https://armypubs.army.mil/epubs/DR_pubs/DR_a/pdf/web/atp5_0x1.pdf.
- OCIO, Army Data Plan, 6.
Maj. Daniel Marcey, U.S. Army, is an Art of War Scholar at the Command and General Staff College. He holds an MA from Liberty University in executive leadership and a BS in civil engineering technology from Old Dominion University. Previous assignments include the 3rd Infantry Division, 1st Armored Division, and the Corps of Engineers’ Engineer Research and Development Center in Vicksburg, Mississippi.
JML Call for Papers
The Journal of Military Learning (JML) is a peer-reviewed semiannual publication that supports the military’s effort to improve education and training for the U.S. Army and the overall profession of arms. The JML invites practitioners, researchers, academics, and military professionals to submit manuscripts that address the issues and challenges of adult education and training such as education technology, adult learning models and theory, distance learning, training development, and other subjects relevant to the field. Book reviews of published relevant works are also encouraged.
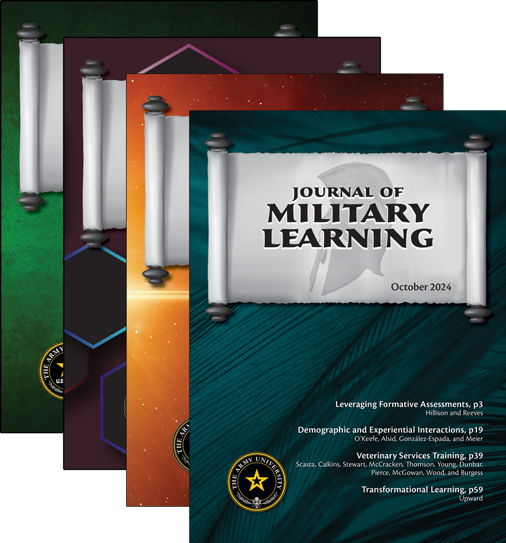
We are now accepting manuscripts for future editions of JML. Manuscripts should be submitted to usarmy.leavenworth.tradoc.mbx.journal-of-military-learning@army.mil. Submissions should be between 3,500 and 5,000 words and supported by research, evident through the citation of sources. For detailed author submission guidelines or to view past or current editions, scan the QR code or visit JML on the Army University Press website at http://www.armyupress.army.mil/Journals/Journal-of-Military-Learning/.
For additional information, send an email to the above address.
Back to Top