Taking a Data-Centric Approach to Unit Readiness
Leveraging Analytics in a Brigade Combat Team
Col. Anthony Keller, U.S. Army
Lt. Col. Jonathan Bate, U.S. Army
Capt. Brendon Wamsley, U.S. Army
Download the PDF 
Over the past two decades, the business world has aggressively leveraged the massive amounts of data available to improve results and efficiency—not doing so risks leaving corporations at a severe competitive disadvantage. When applied properly, data analytics can generate powerful insights that resonate across an organization. For example, in 2009, when Google considered eliminating thousands of managerial positions in favor of a radically flatter organization, it implemented a now-famous multiyear research effort, “Project Oxygen.” Its purpose was to answer the fundamental question of whether managers matter. Using performance reviews and employee survey data, Google learned that teams with higher quality managers did indeed enjoy superior performance and higher employee retention, and reversed course, instead investing in its managers.1 Through further research, it identified eight key behaviors that contributed to managerial effectiveness and has since used this information to systematically train and cultivate its leader talent.
The U.S. Army, in contrast, has not kept pace with advances in data analytics, despite the vast amount of data it collects daily. Analyzing this data could now not only allow it to better measure performance and prioritize resources but also answer fundamental questions such as why soldiers choose to reenlist and what factors can reduce harmful behaviors like suicide and sexual assault. Achieving the Secretary of the Army’s second objective of making the Army more data-centric has the potential to not only help us “prevail on the future battlefield” but also generate combat readiness more efficiently.2 To be effective, this initiative must not only be top-down—grassroots change can begin with leaders at brigade and below.
Data analytics is of itself challenging to implement, but tactical brigades face additional obstacles, particularly lack of expertise. This article offers a framework for overcoming these obstacles to put data analytics to work in support of company through brigade-level commanders. In the summer of 2023, 1st Stryker Brigade Combat Team (1SBCT), 4th Infantry Division (4ID), created a team to leverage its organic data talent, the Raider Analytics, Innovation, and Data (RAID) team. Its goal is to embed analytics into routine staff systems to generate insights to inform commanders so they can achieve better results than they could without leveraging data. Building high-quality datasets will support the 2023 DOD Data, Analytics, and Artificial Intelligence Adoption Strategy, which seeks to increase our “decision advantage” and produce superior results across warfighting functions while enabling development of artificial intelligence systems.3
What Is Data Analytics?
Simply put, data analytics is the process of converting raw data into actionable insights.4 It encompasses a range of software tools and statistical techniques that use data to identify trends and generate insights that can help solve problems. There are four main types of data analytics: descriptive, diagnostic, predictive, and prescriptive.5 Descriptive analytics tells us what happened, diagnostic analytics tell us why it happened, predictive analytics forecasts future results, and prescriptive analytics argues for a decision or action. If used properly, these types of analytics can drive better decisions across organizations.
By discovering previously unnoticed connections between variables, as in Google’s Project Oxygen, data analytics can generate insights that eliminate guesswork, suggest cost-saving techniques, and enable more effective problem-solving. It can also help evaluate unit and program performance. Analyzing data allows us to move up the knowledge management hierarchy from data to wisdom (shown in figure 1).
Turning Data into Decisions: The Raider Data Analytics Process
Army units generate a tremendous amount of data every day, but only a fraction of it is used to drive informed decisions. To solve this problem and implement data analytics in the Raider Brigade, we created the “Raider Data Analytics Process,” shown in figure 2. It is a seven-step process for turning data into insights that can drive leader decisions. Using a framework makes the system more approachable and enduring.
Step 1: Define objectives. First, we must frame the problem we are attempting to solve. This requires asking the right questions to select the correct type of data analytics. For example, “What were trends in the brigade’s fully mission capable (FMC) equipment rates over the past year?” (descriptive), or “Why did Stryker FMC rates drop last month?” (diagnostic). Good questions should be directly related to decisions. If FMC rates dropped significantly, this could indicate a need for greater funding, more leader attention, or changes in preventive maintenance checks and services, for example. The latter question could highlight a specific practice, such as parts ordering, that could improve FMC rates. A substep here is to develop a logic model to show how concepts are related as shown in the figure 3 example proposing a link between unit morale and harmful. The objective could be to identify trends or to test a hypothesis—for example, higher unit morale is correlated with fewer harmful behaviors. Substantive knowledge about underlying processes is critical for designing valuable studies.
Step 2: Gather data. Having defined the research question, we must collect data that can answer this question. We now must now think in terms of variables, which are the “features of the world” that we seek to measure and understand.6 In data analytics, variables are generally divided into three types: explanatory (independent), outcome (dependent), and control.
In this step, we operationalize our proposed logic model by measuring concepts to produce variables. Data does not only consist of numbers; almost anything, including text, can be data if it says something about a feature of the world. When it comes to measuring variables, there are two main types of data: quantitative and qualitative (see figure 4). Quantitative data is either discrete (only whole numbers, such as number of Strykers) or continuous (can take any value between two numbers, such as FMC rates). Qualitative data is nominal (categories without any particular order, such as unit names) or ordinal (different categories with a natural ranked order, such as “red, amber, green”).
Key to this step is correctly measuring variables and structuring the data. The concept of “unit of analysis” is key, as it defines the comparison we are making in accordance with the research question. This requires both a unit of measurement and a time period. For example, we could compare FMC status for individual Strykers or average monthly Stryker FMC rates for battalions/squadrons. Dataset structures fall into three main categories: cross-sectional (multiple unit measurements at a single point in time), time series (measurements of a single unit over multiple time periods), or time-series cross-sectional (also known as “panel” data; multiple unit measurements over multiple time periods). Different data structures allow us to draw different conclusions from the data. A key challenge in data collection is ensuring consistent measurement over time.
Data sources include programs of record, such as Army Vantage and Global Combat Support System-Army (GCSS-A), internal staff trackers, and surveys.7
Step 3: Prepare data. Preparing the data requires understanding the format and accounting for consistent measurement of variables and missing data. It consists of cleaning and reformatting as necessary, which can be done in Microsoft Excel or in statistical software packages such as R Studio.
Step 4: Visualize data. Visualization provides a graphical representation of the data, which allows us to visually inspect trends and make comparisons. Doing helps us understand the distribution and structure of the data, which can provide insights by itself or guide the type of analysis we pursue.
Step 5: Analyze data. Data analysis is the critical step to generating insights. We can move from descriptive statistics, correlation analysis, and causal inference. Descriptive statistics show us the distribution and spread of the data. Arithmetic means (commonly known as averages) can hide a large amount of variation in the data. Correlation analysis looks for statistically significant relationships (not occurring due to chance) between two or more variables (keeping in mind that correlation does not equal causation). Causal inference suggests that one variable causes another. Determining causality requires identifying (or simulating) separate groups that did and did not receive some type of “treatment” (e.g., treatment and control groups).
The tools required to analyze data are readily available at low cost. Microsoft Excel, readily available on government computers, provides a range of data visualization and analysis. More advanced statistical software such as R and Python expand the options. Linear regression analysis is the “workhorse” in determining correlations (though there are many other types). It is a statistical technique for testing whether changes observed in the outcome (dependent) variable are associated with changes in one or more of the explanatory (independent) variables. It does this by fitting a straight line (a very simple data model) through data points. Regression results provide coefficients on each explanatory and control variable. The magnitude and statistical significance of these coefficients communicates the strength of the relationship in the mathematical model.
Step 6: Interpret results. Interpreting results can be difficult. Whether analysis consisted of descriptive statistics, correlation analysis, or causal inference, it is critical to put the results in context. For example, statistically significant coefficients in a linear regression tell us the “predicted” change in the outcome variable, given incremental changes in the explanatory variables. Interpreting data requires experience and qualitative knowledge. Statistics can easily mislead, so experience and intuition are critical for putting quantitative results in context.
Step 7: Communicate results. Communicating results is key to enabling data-informed decisions. It must focus on the “so what” of the results that produce insights that can enable decisions. We have done this through single PowerPoint slides accompanied by short information papers distributed to command teams and staff.
Turning Data into Decisions: Raider Brigade Use Cases
During its first six months of operation, the RAID Team pursued questions in the areas of harmful behaviors, unit readiness, and unit culture. It used both existing data and generated data using a unit survey. The four resulting use cases highlight the power of data analytics to produce actionable insights.
Case 1: Harmful behaviors. The team examined patterns in soldier harmful behaviors, measured by the number of serious incident reports (SIRs) in four categories: DUIs, violent events, weapon offenses, and suicide events. The project asked whether recent patterns of harmful behaviors at the company/troop/battery (CTB) level are associated with increased probability of SIRs in the current week—in other words, do units experience “surges” in SIRs? SIR data is compiled by the brigade provost martial officer. Using this dataset (ranging the previous two years for thirty-seven CTBs), we addressed the research question by comparing the probability of an SIR in CTBs in the current week, when considering how many SIRs they experienced in the past three weeks. A linear data model called a logistic regression enabled this analysis.
We found that a CTB that experienced three SIRs in the past three weeks had at least a 20 percent greater chance of an SIR in the current week. Identifying this “momentum effect” in SIR trends enabled creation of the 4th Infantry Division Unit Risk Forecasting Tool that uses Power Automate to send automated alerts enabling command teams to diagnose underlying unit issues.8 With this information, they can better focus “care” resources (unit ministry teams, behavioral health, etc.) on units that need them most to break the cycle of SIRs in CTBs.9
Case 2: Stryker maintenance. This project originated from a forward support company (FSC) commander’s insightful question about whether work order turnaround time (TAT) is related to ERC-P (equipment readiness code-pacing item) FMC rates. He suspected that lower TAT is associated with higher Stryker FMC rates. This question matters because it could shed light on indicators of effective maintenance. Nearly five years of GCSS-A work order and equipment FMC rate data allowed us to answer this question using linear regression.
We found that average monthly TAT is negatively correlated with Stryker FMC rates at the battalion/squadron level. The relationship is relatively small in magnitude (between 0.05 and 0.17 percentage points of FMC rate per average day of TAT), but statistically robust, suggesting that additional factors (such as funding, operational tempo, etc.) may provide greater explanatory value.10 We are actively working to discover these missing variables to improve our model. The main takeaway of this project is that commanders should focus on TAT as a more valuable metric than FMC rates, since units can more directly influence it by ensuring work orders are closed promptly.
Case 3: Unit culture. Applying data analytics to unit culture and climate is difficult because of their intangible nature. We tackled this challenge by generating data on seven culture “measurables” using a short cell phone survey taken by all soldiers every two months. The results have helped leaders identify strengths and weaknesses in their units across areas such as care for soldiers, information flow, and leader competence.11
Analysis of low scores in specific areas—supplemented by qualitative methods that include soldier interviews by chaplains—allows command teams to take action to remedy potential problems. Additionally, the unit culture data allowed us to identify relationships that help explain key outcomes. For example, soldier professional development is positively correlated with higher retention, while unit inspiration is linked with fewer SIRs.
Case 4: Stryker gunnery. Data analytics can help improve unit lethality by identifying key factors that leaders can influence. Inspired by Billy Beane’s “Moneyball” approach to improving baseball performance, we asked whether data analytics could help us identify the factors that improve crew performance during Stryker mounted machine gun engagements. We found that like baseball, in-game statistics during gunnery can identify factors that correlate with better crew performance. Using data from the brigade’s Stryker mounted machine gun qualification firing, we found that Table III qualification scores can help forecast the probability that a crew will qualify at the final Table VI on the first attempt (“Q1”).12 This result allowed us to estimate a minimum threshold score on Table III that crews need to have a 90 percent chance of achieving a Q1 rating. If a crew does not achieve this threshold, command teams can have them return to earlier gunnery tables for retraining, saving ammunition while improving results. Additionally, we found a robust correlation between stronger unit culture and better crew performance during Table VI, suggesting that engaged leadership is a key component of unit lethality.
The Way Ahead: Data Lakes, Literacy, and Leadership
Implementing data analytics in a BCT is not easy. The three main challenges are data architecture, expertise, and culture—put more simply, data “lakes,” literacy, and leadership.13
First, although Army units generate massive amounts of data, it is not always accessible, organized, or properly formatted. Effective data management is critical to modeling that supports decisions. This involves turning isolated, disconnected data “pools” into much larger data “lakes.” To enable data analysis, we must organize available data streams to build properly formatted datasets. The seven DOD data principles, first laid out in the 2020 DOD Data Strategy, are valuable—data should be visible, accessible, understandable, linked, trustworthy, interoperable, and secure.14 However, Army data is often not arrayed in a way that it is directly usable. For example, GCSS-A organizes maintenance data by battalion “work center” identifiers, whereas our Stryker maintenance project described earlier requires the maintenance data to be tied to units, meaning we need unit names or unit identification codes (UICs) to be present in the dataset—a very simple problem that can pose a major roadblock without sufficient time or data skills required to wrangle the dataset.
Second, BCTs often lack formal expertise in data, not typically being assigned an operations research/systems analyst. Learning to code and perform statistical analysis is an obstacle. Although data literacy is a key skill, it is lacking in our formations. There is typically unexploited talent of all ranks across tactical formations that leaders can develop and empower. Free online resources and unit data literacy training events (such as the 4th Infantry Division “Ivy Data Academy”) are productive ways of increasing data skills.
Finally, leadership is essential. To produce data-centric units, senior leaders must foster a culture that values evidence-based decision-making. Embedding data analysis into daily operations requires that commanders “normalize bottom-up problem-solving, especially when dealing with data issues.”15 By providing a “demand signal” for the insights that data can provide and resourcing the analytical efforts, commanders can empower soldiers at all echelons to leverage data science to solve problems.
The Raider Brigade addressed all three obstacles by creating a data analytics team led by a battalion commander with data science skills. The brigade commander empowered the team and integrated the insights it generated into his decision-making. Leaders across the brigade shared these insights by publishing papers in coordination with the Harding Project.
Toward Decision Dominance
Data analytics offers accessible, valuable tools for generating insights from data at all echelons. While challenges exist, we can overcome them with effective data management, literacy training, and leadership. Brigade and battalion staffs harnessing the power of data analytics is critical to helping the Army become data-centric from the ground up. The information advantage that it can create will move us toward decision dominance—faster, better decisions that help commanders win on the battlefield.16
Notes 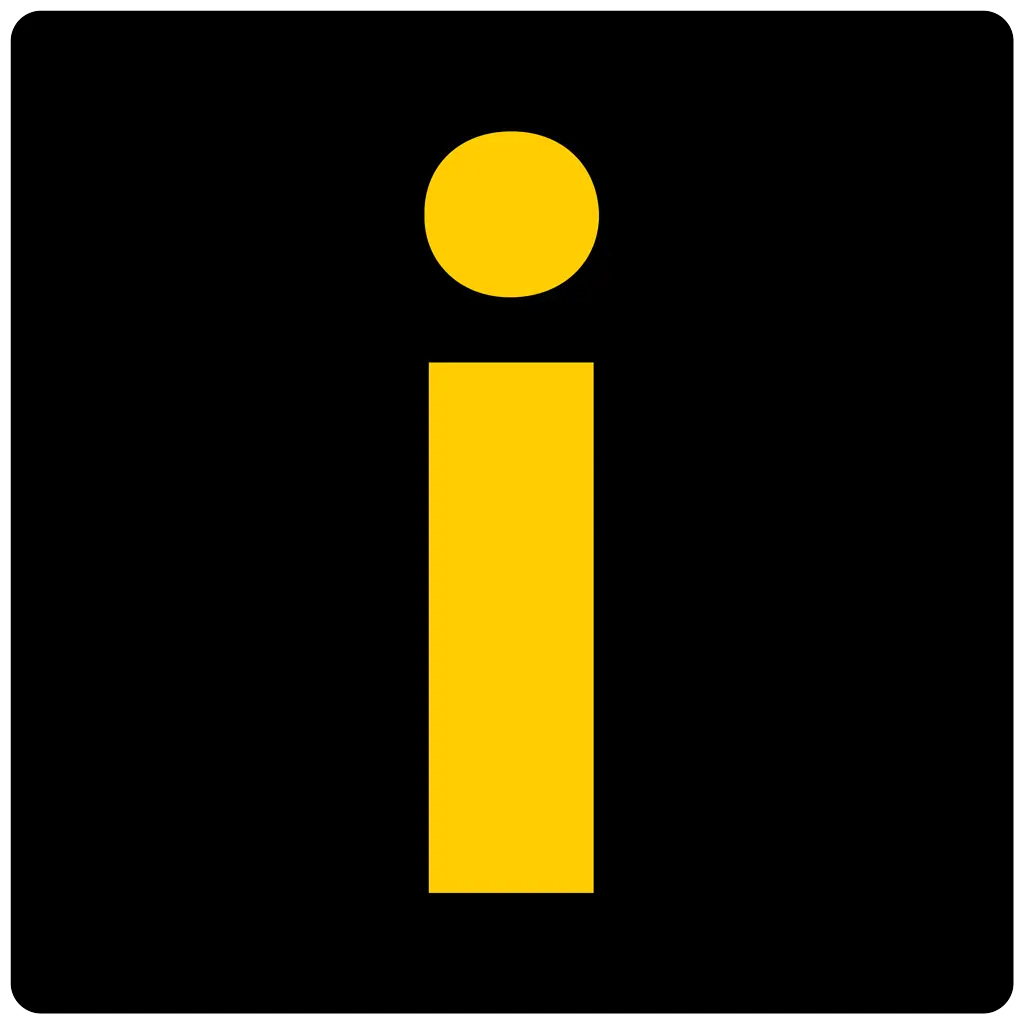
- David Garvin, “How Google Sold Its Engineers on Management,” Harvard Business Review (website), December 2013, https://hbr.org/2013/12/how-google-sold-its-engineers-on-management.
- Christine E. Wormuth, “Message from the Secretary of the Army to the Force,” Army.mil, 8 February 2022, https://www.army.mil/article/253814/message_from_the_secretary_of_the_army_to_the_force.
- Chief Digital and Artificial Intelligence Office, “2023 Data, Analytics, and Artificial Intelligence Adoption Strategy: Accelerating Decision Advantage,” U.S. Department of Defense, 2 November 2023, https://media.defense.gov/2023/Nov/02/2003333301/-1/-1/1/DAAIS_FACTSHEET.PDF.
- “What Is Data Analytics?,” Amazon Web Services, accessed 21 October 2024, https://aws.amazon.com/what-is/data-analytics/.
- Iqbal H. Sarker, “Data Science and Analytics: An Overview from Data-Driven Smart Computing, Decision-Making and Applications Perspective,” SN Computer Science 2, no. 5 (2021): 377, https://www.ncbi.nlm.nih.gov/pmc/articles/PMC8274472/#Sec8.
- Ethan Bueno de Mesquita and Anthony Fowler, Thinking Clearly with Data: A Guide to Quantitative Reasoning and Analysis (Princeton, NJ: Princeton University Press, 2021), 13.
- Army Vantage is a cloud-based, data-driven platform run by Palantir that helps the U.S. Army make decisions about a range of areas, including personnel, equipment, and training. Global Combat Support System—Army (GCSS-A) is a web-based system that manages the U.S. Army’s logistics and finances. It’s designed to improve the Army’s ability to track supplies, maintain accountability for property, monitor maintenance, and report on assets.
- Lauren Williams, “How One Army Infantry Division Is Using Data Analytics to Keep Soldiers Safe,” Defense One, 20 August 2024, https://www.defenseone.com/defense-systems/2024/08/how-one-army-infantry-division-using-data-analytics-keep-soldiers-safe/398949/.
- Taylor Graham, “The Project Prevention Journey: Raider Analytics, Innovation, and Data Team (RAID-T) Efforts to Forecast and Reduce Harmful Behaviors,” Army.mil, 18 April 2024, https://www.army.mil/article/275437/the_project_prevention_journey_raider_analytics_innovation_and_data_team_raid_t_efforts_to_forecast_and_reduce_harmful_behaviors.
- Moving from an average turnaround time of one hundred to fifty days is associated with a fully mission-capable rate that is roughly 0.5 percent points higher (from 89.5 to 90.0, for example).
- Josh Bowen and Jon Bate, “Cohesion, Performance, and Readiness: A Brigade-Level Experiment in the Art and Science of Organizational Culture,” Modern War Institute, 29 March 2024, https://mwi.westpoint.edu/cohesion-performance-and-readiness-a-brigade-level-experiment-in-the-art-and-science-of-organizational-culture/.
- Lauren Williams, “‘Moneyball’ for Gun Crews: Surprising Data Have Army Division Reshaping Its Gunnery Training,” Defense One, 1 September 2024, https://www.defenseone.com/defense-systems/2024/09/moneyball-gun-crews-surprising-data-have-army-division-reshaping-its-gunnery-training/399227/.
- Jonathan Bate and Nathan Platz, “Harnessing the Power of Data,” Association of the United States Army, 4 September 2024, https://www.ausa.org/articles/harnessing-power-data.
- Department of Defense, Executive Summary: DoD Data Strategy (Washington, DC: Department of Defense, 30 September 2020), https://media.defense.gov/2020/Oct/08/2002514180/-1/-1/0/DOD-DATA-STRATEGY.PDF.
- Orlando Nieves III, John Boyer, and Feihrren Calhoun, “Take Ownership of Your Formation’s Data Literacy,” Military Review Online Exclusive, 25 August 2023, https://www.armyupress.army.mil/journals/military-review/online-exclusive/2023-ole/data-literacy/.
- Karl Gingrich and Bryan Shone, “Enhance Decisions with Data,” Association of the United States Army, 24 October 2022, https://www.ausa.org/articles/enhance-decisions-data.
Col. Anthony Keller, U.S. Army, commands 1st Stryker Brigade Combat Team, 4th Infantry Division. He received his commission from the Citadel, the Military College of South Carolina, in 2002, and continued his education at the Naval War College and the Naval Postgraduate School. He previously served in the 25th Infantry Division; the 75th Ranger Regiment; the 101st Airborne Division; the 2nd Cavalry Regiment; the Joint Interagency Task Force–National Capital Region; the 82nd Airborne Division; and as chief of operations, G-3/5/7-OD, in Headquarters, Department of the Army.
Lt. Col. Jonathan Bate, U.S. Army, commands 2nd Battalion, 23rd Infantry Regiment, 1st Stryker Brigade Combat Team, 4th Infantry Division. He also leads the brigade’s grassroots data analytics team. He previously served in the 101st Airborne Division, the 1st Armored Division , and as assistant professor of economics in the U.S. Military Academy Department of Social Sciences. A Goodpaster Scholar in the Advanced Strategic Planning and Policy Program, Bate holds an MPP from the Harvard Kennedy School and PhD in political science from Stanford University.
Capt. Brendon Wamsley, U.S. Army, served five years as an active-duty military police officer. He currently works as a client executive at BlueCloud, a leading solutions provider in the tech industry. He holds a BS in economics from the U.S. Military Academy and an MA in business and organizational security management.
Back to Top